Using Accelerate to speed up PyTorch model training
Accelerate is a powerful tool developed by Hugging Face that’s designed to manage distributed training across multiple CPUs, GPUs, and TPUs, or even cloud services such as Amazon Web Services (AWS), Google Cloud Platform (GCP), and Microsoft Azure. It abstracts data and model parallelism and efficiently distributes computations across multiple CPUs, GPUs, or TPUs, reducing overhead and streamlining execution, thereby making scaling effortless. The best part is that you need to add just five lines of accelerate
code into the existing PyTorch code to optimally utilize the hardware, as shown in Figure 19.6:
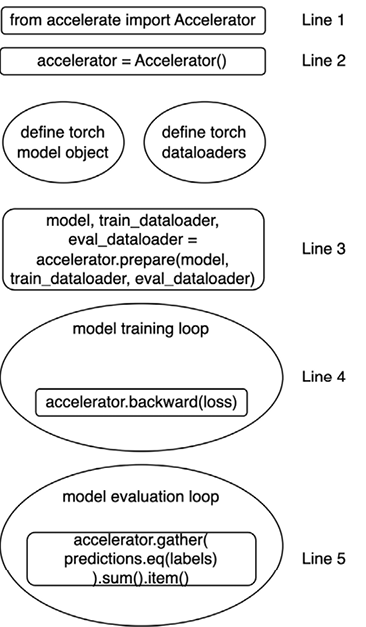
Figure 19.6: Schematic representation of accelerating PyTorch model training code with just five lines of accelerate code
To illustrate the usage of accelerate
, we will continue the previous example of fine-tuning a BERT model for text classification and use accelerate inside the training code to optimize the...