To stay ahead of the times and take informed decisions, businesses now require running analytics on data that is moved in on a real-time basis and this data is usually multi-structured, characterized in the previous section. Value is in identifying patterns to make intelligent decisions and in influencing decisions if we could see the behavior patterns.
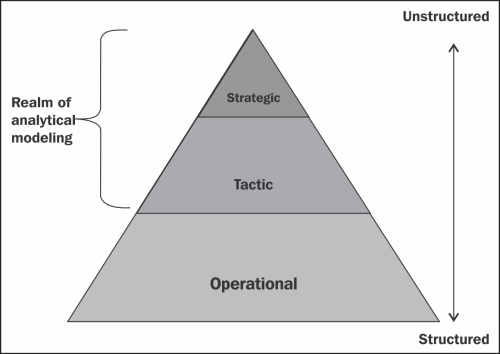
Classically, there are three major levels of management and decision making within an organization: operational, tactical, and strategic. While these levels feed one another, they are essentially distinct:
Operational data: It deals with day-to- day operations. At this level decisions are structured and are usually driven by rules.
Tactical data: It deals with medium-term decisions and is semi-structured. For example, did we meet our branch quota for new loans this week?
Strategic data: It deals with long-term decisions and is more unstructured. For example, should a bank lower its minimum balances to retain more customers and acquire more new customers?
Decision making changes as one goes from level to level.
With increasing need for supporting various aspects of Big Data, as stated previously, existing data warehousing and business intelligence tools are going through transformation.
Big Data is not, of course, just about the rise in the amount of data we have, it is also about the ability we now have to analyze these data sets. It is the development with tools and technologies, including such things as Distributed Files Systems (DFS), which deliver this ability.
High performance continues to be a critical success indicator for user implementations in Data Warehousing (DW), Business Intelligence (BI), Data Integration (DI), and analytics. Advanced analytics includes techniques such as predictive analytics, data mining, statistics, and Natural Language Processing (NLP).
A few important drivers for analytics are listed as follows:
Need to optimize business operations/processes
Proactively identify business risks
Predict new business opportunities
Compliance to regulations
Big Data analytics is all about application of these advanced analytic techniques to very large, diverse data sets that are often multi-structured in nature. Traditional data warehousing tools do not support the unstructured data sources and the expectations on the processing speeds for Big Data analytics. As a result, a new class of Big Data technology has emerged and is being used in many Big Data analytics environments. There are both open source and commercial offerings in the market for this requirement.
The focus of this book will be Greenplum UAP that includes database (for structured data requirements), HD/Hadoop (for unstructured data requirements), and Chorus (a collaboration platform that can integrate with partner BI, analytics, and visualization tools gluing the communication between the required stakeholders).
The following diagram depicts the evolution of analytics, very clearly, with the increase in data volumes; a linear increase in sophistication of insights is sought.
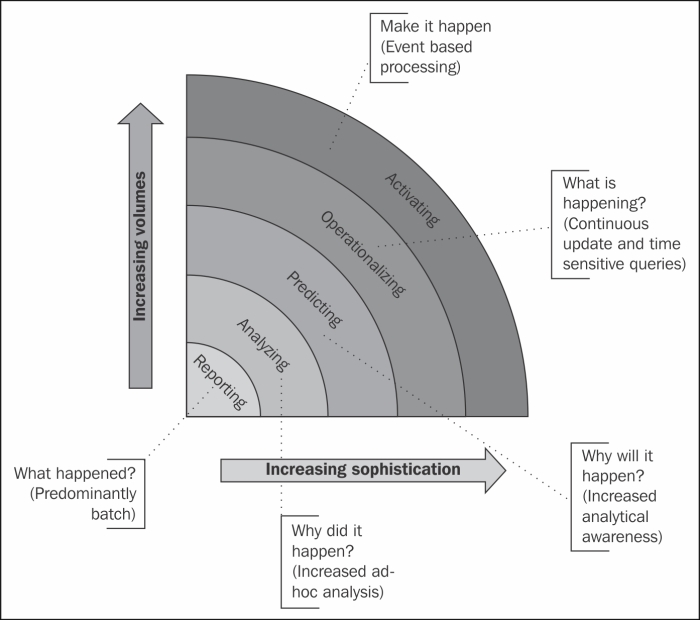
Initially, it was always Reporting. Data was pre-processed and loaded in batches, and an understanding of "what happened?" was gathered.
Focus slowly shifted on to understanding "why did it happen?". This is with the advent of increased ad-hoc data inclusion.
At the next level, the focus has shifted to identifying "why will it happen?", a focus more on prediction instead of pure analysis.
With more ad-hoc data availability, the focus is shifted onto "what is happening?" part of the business.
Final focus is on "make it happen!" with the advent of real-time event access.
With this paradigm shift, the expectations from a new or modern data warehousing system have changed and the following table lists the expected features:
Challenges |
Traditional analytics approach |
New analytics approach |
---|---|---|
Scalability |
N |
Y |
Ingest high volumes of data |
N |
Y |
Data sampling |
Y |
N |
Data variety support |
N |
Y |
Parallel data and query processing |
N |
Y |
Quicker access to information |
N |
Y |
Faster data analysis (higher GB/sec rate) |
N |
Y |
Accuracy in analytical models |
N |
Y |
A few of the analytical techniques we will be further understanding in the following chapters are:
Descriptive analytics: Descriptive analytics provides detail on what has happened, how many, how often, and where. In this technique, new insights are developed using probability analysis, trending, and development of association over data that is classified and categorized.
Predictive analytics: Predictive modeling is used to understand causes and relationships in data in order to predict valuable insights. It provides information on what will happen, what could happen, and what actions can be taken. Patterns are identified in the data using mathematical, statistical, or visualization techniques. These patterns are applied on the new data sets to predict the behavior.
Prescriptive analytics: Prescriptive analytics helps derive a best possible outcome by analyzing the possible outcomes. It includes Descriptive and Predictive analytic techniques to be applied together. Probabilistic and Stochastic methods such as Monte Carlo simulations and Bayesian models to help analyze best course of action based on "what-if" analysis.