Now that we have built our first decision tree, it's time to turn our attention to a real dataset: the Breast Cancer Wisconsin dataset (https://archive.ics.uci.edu/ml/datasets/Breast+Cancer+Wisconsin+(Diagnostic)).
This dataset is a direct result of medical imaging research, and is considered a classic today. The dataset was created from digitized images of healthy (benign) and cancerous (malignant) tissues. Unfortunately, I wasn't able to find any public-domain examples from the original study, but the images look similar to the following one:
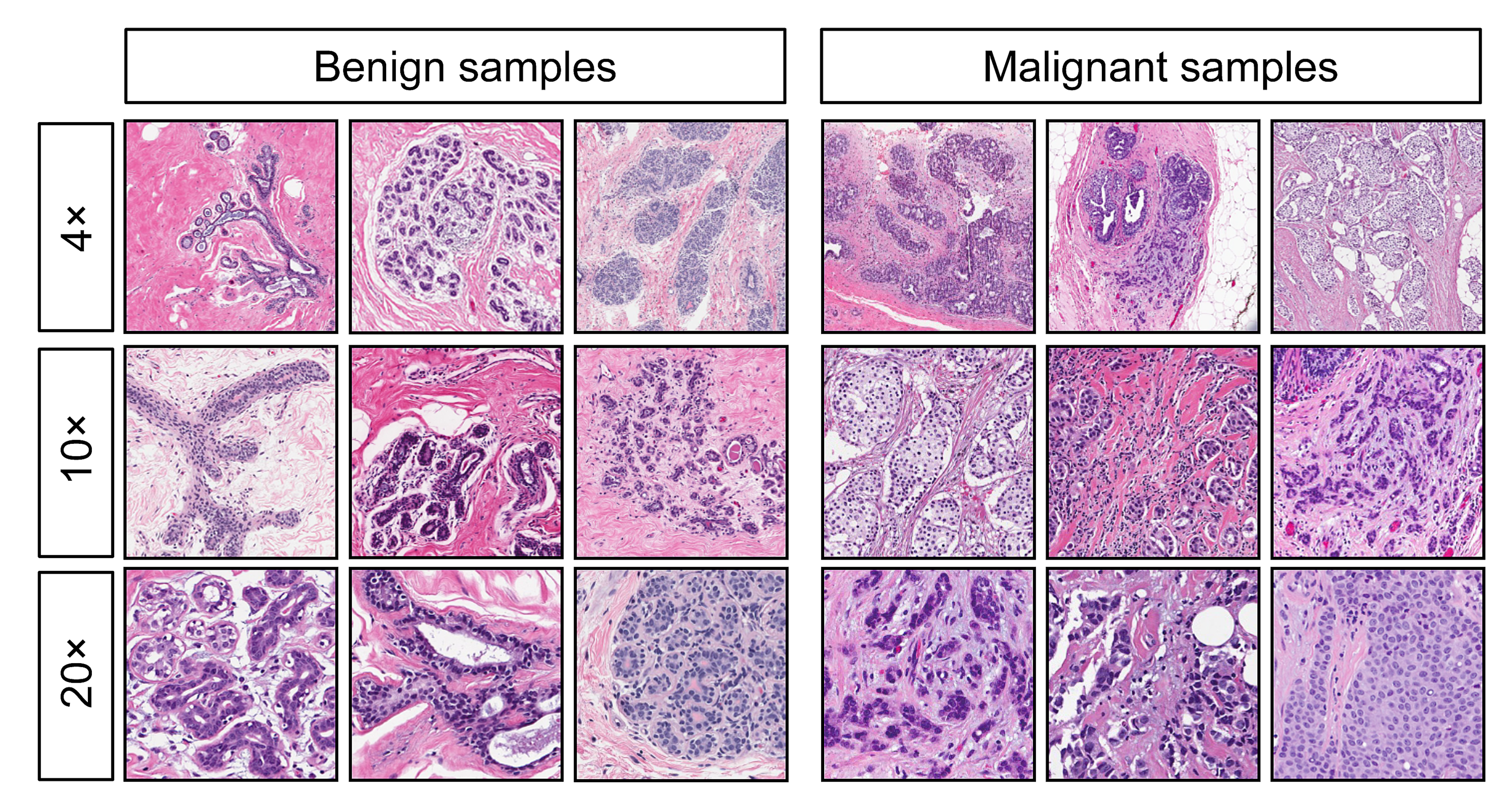
Breast cancer tissue samples from Levenson et al. (2015), PLOS ONE, doi:10.1371/journal.pone.0141357. Released under CC-BY.
The goal of the research was to classify tissue samples into benign and malignant (a binary classification task).
In order to make the classification task feasible...