There are several terms that we must define before discussing how principal component analysis works.
Recall that variance is a measure of how a set of values are spread out. Variance is calculated as the average of the squared differences of the values and mean of the values, as per the following equation:
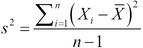
Covariance is a measure of how much two variables change together; it is a measure of the strength of the correlation between two sets of variables. If the covariance of two variables is zero, the variables are uncorrelated. Note that uncorrelated variables are not necessarily independent, as correlation is only a measure of linear dependence. The covariance of two variables is calculated using the following equation:
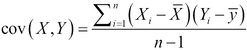
If the covariance is nonzero, the sign indicates whether the variables are positively or negatively correlated. When two variables are positively correlated, one increases as the other increases...