All right. You have arrived at the last section of exploratory data analysis. Now you will expand your exploration to multiple variables at once. Typical datasets have many variables, but a bivariate analysis limits you to pairwise comparisons. Exploring five variables, two at a time creates 10 pairs, 10 variables create 45, 20 variables create 190, 40 variables create 780, and so on. The impact on workflow is nearly exponential, as shown in the following diagram:
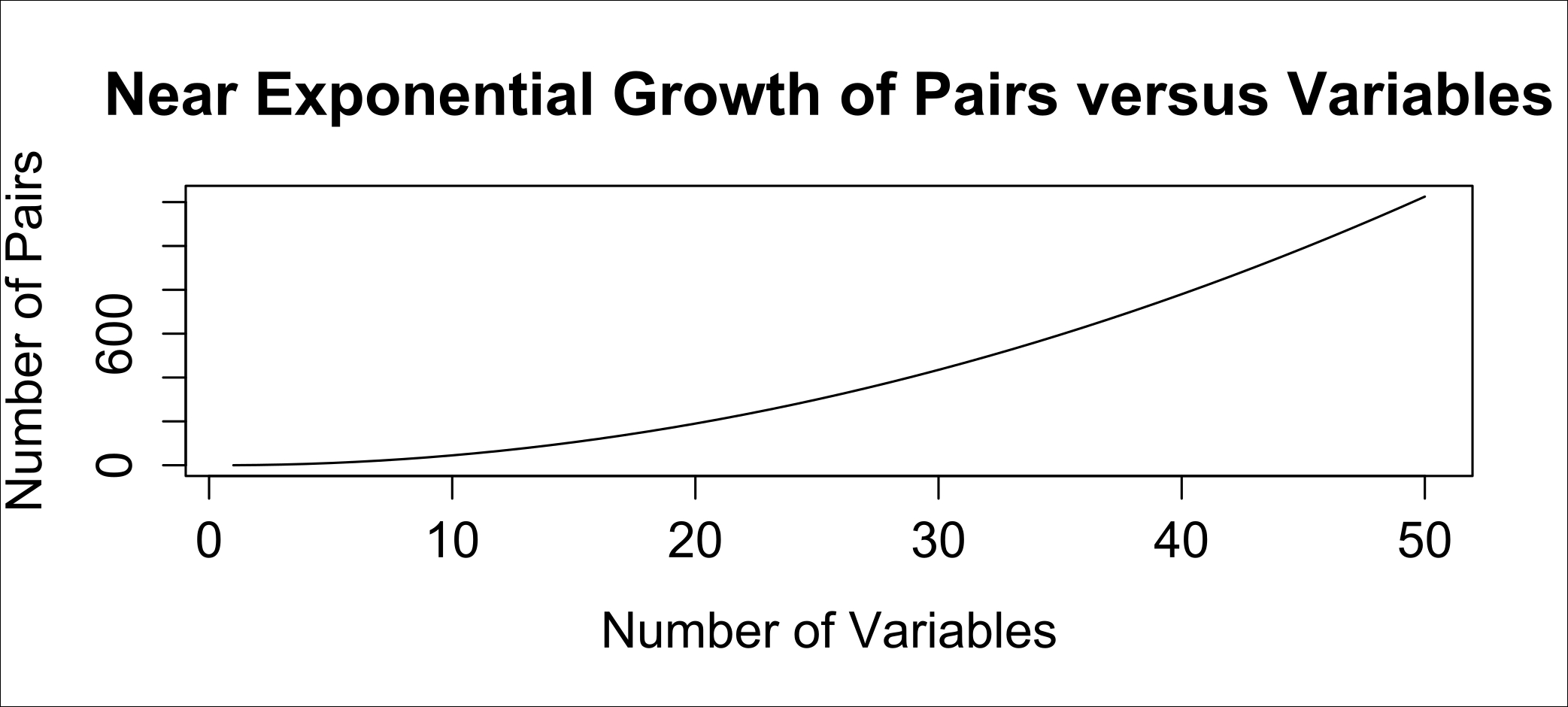
As the number of features (variables) in your dataset grows, your strategy for exploratory data analysis must scale along with your data. Your knowledge of bivariate exploratory data analysis provides you the following two benefits:
You have the foundations for exploring multiple variables simultaneously
You can use bivariate analysis to further explore any interesting pairs
You will still use the four-question approach of Look-Relationships-Correlation-Significance.