Any effort to apply machine learning to a large-sized problem requires the collaborative effort of a number of roles, each abiding by a set of systematic processes designed for rigor, efficiency, and robustness. The following roles and processes ensure that the goals of the endeavor are clearly defined at the outset and the correct methodologies are employed in data analysis, data sampling, model selection, deployment, and performance evaluation—all as part of a comprehensive framework for conducting analytics consistently and with repeatability.
Participants play specific parts in each step. These responsibilities are captured in the following four roles:
Business domain expert: A subject matter expert with knowledge of the problem domain
Data engineer: Involved in the collecting, transformation, and cleaning of the data
Project manager: Overseer of the smooth running of the process
Data scientist or machine learning expert: Responsible for applying descriptive or predictive analytic techniques
CRISP (Cross Industry Standard Process) is a well-known high-level process model for data mining that defines the analytics process. In this section, we have added some of our own extensions to the CRISP process that make it more comprehensive and better suited for analytics using machine learning. The entire iterative process is demonstrated in the following schematic figure. We will discuss each step of the process in detail in this section.
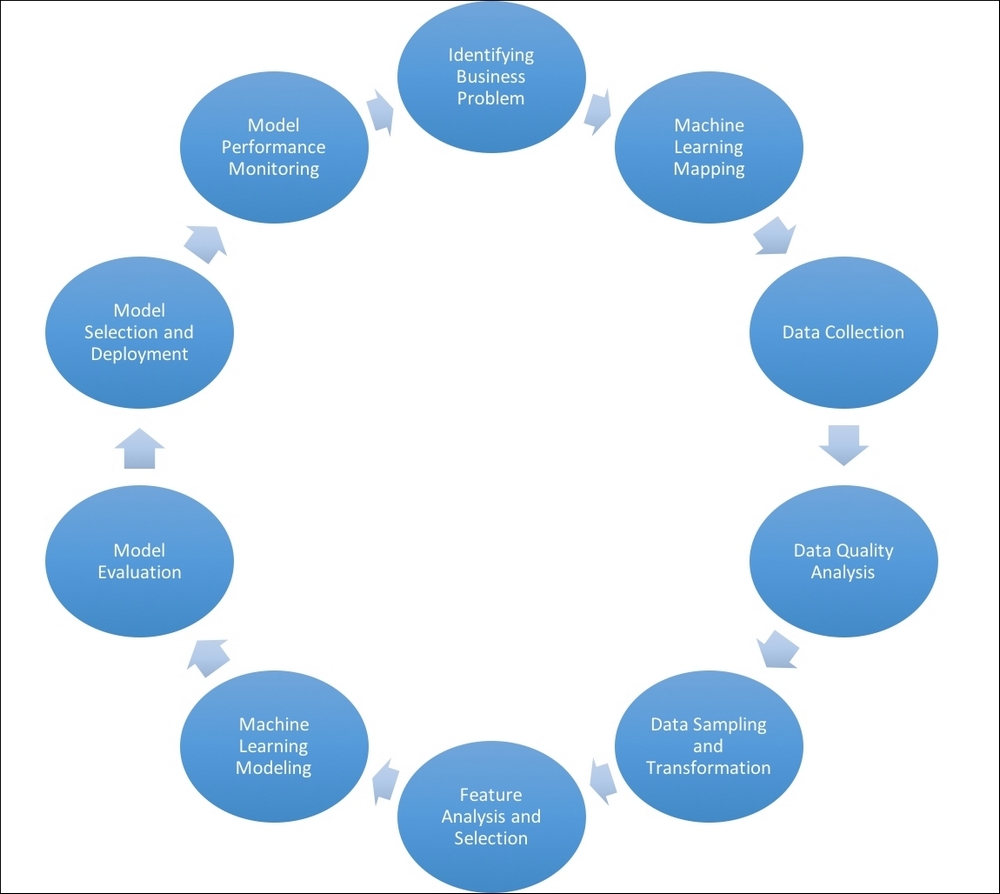
Identifying the business problem: Understanding the objectives and the end goals of the project or process is the first step. This is normally carried out by a business domain expert in conjunction with the project manager and machine learning expert. What are the end goals in terms of data availability, formats, specification, collection, ROI, business value, deliverables? All these questions are discussed in this phase of the process. Identifying the goals clearly, and in quantifiable terms where possible, such as dollar amount saved, finding a pre-defined number of anomalies or clusters, or predicting no more than a certain number of false positives, and so on, is an important objective of this phase.
Machine learning mapping: The next step is mapping the business problem to one or more machine learning types discussed in the preceding section. This step is generally carried out by the machine learning expert. In it, we determine whether we should use just one form of learning (for example, supervised, unsupervised, semi-supervised) or if a hybrid of forms is more suitable for the project.
Data collection: Obtaining the raw data in the agreed format and specification for processing follows next. This step is normally carried out by data engineers and may require handling some basic ETL steps.
Data quality analysis: In this step, we perform analysis on the data for missing values, duplicates, and so on, conduct basic statistical analysis on the categorical and continuous types, and similar tasks to evaluate the quality of data. Data engineers and data scientists can perform the tasks together.
Data sampling and transformation: Determining whether data needs to be divided into samples and performing data sampling of various sizes for training, validation, or testing—these are the tasks performed in this step. It consists of employing different sampling techniques, such as oversampling and random sampling of the training datasets for effective learning by the algorithms, especially when the data is highly imbalanced in the labels. The data scientist is involved in this task.
Feature analysis and selection: This is an iterative process combined with modeling in many tasks to make sure the features are analyzed for either their discriminating values or their effectiveness. It can involve finding new features, transforming existing features, handling the data quality issues mentioned earlier, selecting a subset of features, and so on ahead of the modeling process. The data scientist is normally assigned this task.
Machine learning modeling: This is an iterative process working on different algorithms based on data characteristics and learning types. It involves different steps, such as generating hypotheses, selecting algorithms, tuning parameters, and getting results from evaluation to find models that meet the criteria. The data scientist carries out this task.
Model evaluation: While this step is related to all the preceding steps to some degree, it is more closely linked to the business understanding phase and machine learning mapping phase. The evaluation criteria must map in some way to the business problem or the goal. Each problem/project has its own goal, whether that be improving true positives, reducing false positives, finding anomalous clusters or behaviors, or analyzing data for different clusters. Different techniques that implicitly or explicitly measure these targets are used based on learning techniques. Data scientists and business domain experts normally take part in this step.
Model selection and deployment: Based on the evaluation criteria, one or more models—independent or as an ensemble—are selected. The deployment of models normally needs to address several issues: runtime scalability measures, execution specifications of the environment, and audit information, to name a few. Audit information that captures the key parameters based on learning is an essential part of the process. It ensures that model performance can be tracked and compared to check for the deterioration and aging of the models. Saving key information, such as training data volumes, dates, data quality analysis, and so on, is independent of learning types. Supervised learning might involve saving the confusion matrix, true positive ratios, false positive ratios, area under the ROC curve, precision, recall, error rates, and so on. Unsupervised learning might involve clustering or outlier evaluation results, cluster statistics, and so on. This is the domain of the data scientist, as well as the project manager.
Model performance monitoring: This task involves periodically tracking the model performance in terms of the criteria it was evaluated against, such as the true positive rate, false positive rate, performance speed, memory allocation, and so on. It is imperative to measure the deviations in these metrics with respect to the metrics between successive evaluations of the trained model's performance. The deviations and tolerance in the deviation will give insights into repeating the process or retuning the models as time progresses. The data scientist is responsible for this stage.
As may be observed from the preceding diagram, the entire process is an iterative one. After a model or set of models has been deployed, business and environmental factors may change in ways that affect the performance of the solution, requiring a re-evaluation of business goals and success criteria. This takes us back through the cycle again.