Yarowsky, D (1995). Unsupervised word sense disambiguation rivaling supervised methods. Proceedings of the 33rd Annual Meeting of the Association for Computational Linguistics (pp. 189–196)
Blum, A., and Mitchell, T (1998). Combining labeled and unlabeled data with co-training. COLT: Proceedings of the Workshop on Computational Learning Theory.
Demiriz, A., Bennett, K., and Embrechts, M (1999). Semi-supervised clustering using genetic algorithms. Proceedings of Artificial Neural Networks in Engineering.
Yoshua Bengio, Olivier Delalleau, Nicolas Le Roux (2006). Label Propagation and Quadratic Criterion. In Semi-Supervised Learning, pp. 193-216
T. Joachims (1998). Transductive Inference for Text Classification using Support Vector Machines, ICML.
B. Settles (2008). Curious Machines: Active Learning with Structured Instances. PhD thesis, University of Wisconsin–Madison.
D. Angluin (1988). Queries and concept learning. Machine Learning, 2:319–342.
D. Lewis and W. Gale (1994). A sequential...
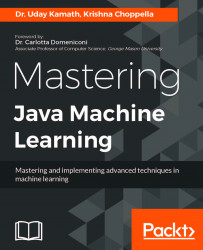
Mastering Java Machine Learning
By :
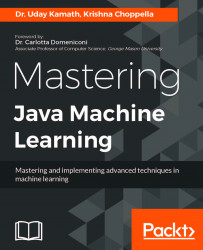
Mastering Java Machine Learning
By:
Overview of this book
Java is one of the main languages used by practicing data scientists; much of the Hadoop ecosystem is Java-based, and it is certainly the language that most production systems in Data Science are written in. If you know Java, Mastering Machine Learning with Java is your next step on the path to becoming an advanced practitioner in Data Science.
This book aims to introduce you to an array of advanced techniques in machine learning, including classification, clustering, anomaly detection, stream learning, active learning, semi-supervised learning, probabilistic graph modeling, text mining, deep learning, and big data batch and stream machine learning. Accompanying each chapter are illustrative examples and real-world case studies that show how to apply the newly learned techniques using sound methodologies and the best Java-based tools available today.
On completing this book, you will have an understanding of the tools and techniques for building powerful machine learning models to solve data science problems in just about any domain.
Table of Contents (20 chapters)
Mastering Java Machine Learning
Credits
Foreword
About the Authors
About the Reviewers
www.PacktPub.com
Customer Feedback
Preface
Machine Learning Review
Practical Approach to Real-World Supervised Learning
Unsupervised Machine Learning Techniques
Semi-Supervised and Active Learning
Real-Time Stream Machine Learning
Probabilistic Graph Modeling
Deep Learning
Text Mining and Natural Language Processing
Big Data Machine Learning – The Final Frontier
Linear Algebra
Probability
Index
Customer Reviews