An SGD implementation of gradient descent uses a simple distributed sampling of the data examples. Loss is a part of the optimization problem, and therefore, is a true sub-gradient.
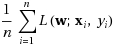
This requires access to the full dataset, which is not optimal.

The parameter miniBatchFraction specifies the fraction of the full data to use. The average of the gradients over this subset
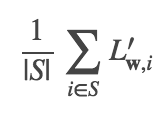
is a stochastic gradient. S is a sampled subset of size |S|= miniBatchFraction.
In the following code, we show how to use stochastic gardient descent on a mini batch to calculate the weights and the loss. The output of this program is a vector of weights and loss.
object SparkSGD {
def main(args: Array[String]): Unit = {
val m = 4
val n = 200000
val sc = new SparkContext("local[2]", "")
val points = sc.parallelize(0 until m,
2).mapPartitionsWithIndex { (idx, iter) =>
val random...