The name of this algorithm comes from the k clusters into which the samples are divided, and the fact that each cluster is grouped around some mean value, a centroid of a cluster. This centroid serves as a prototype of a class. Each data point belongs to the cluster which centroid is the closest.
The algorithm was invented in 1957 at Bell Labs.
In this algorithm, each data point belongs to only one cluster. As a result of this algorithm, we get the feature space partitioned into Voronoi cells.
Note
Because of the k in its name, this algorithm is often confused with the KNN algorithm, but as we already have seen with k-fold cross-validation, not all ks are the same. You may wonder why machine learning people are so obsessed with this letter that they put it in every algorithm's name. I don't k-now.
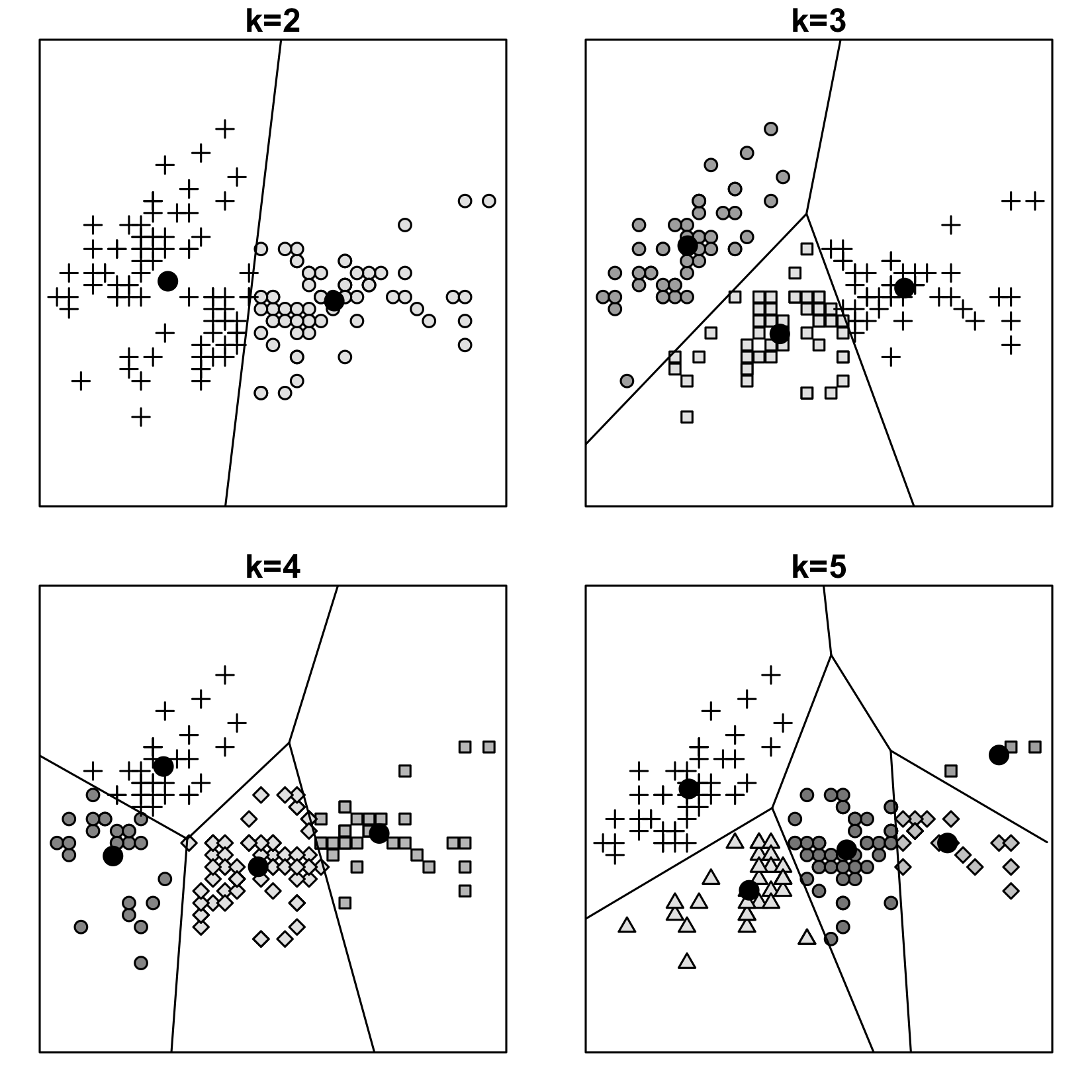
Figure 4.1: Four different ways to cluster the same data using k-means algorithm. Bald black dots are centroids of clusters. The samples are from the classical Iris dataset, plotted...