Welcome! Let's get started. Python has become one of the de facto standard language and platform for data analysis and data science. The mind map that you will see shortly depicts some of the numerous libraries available in the Python ecosystem that are used by data analysts and data scientists. NumPy, SciPy, Pandas, and Matplotlib libraries lay the foundation of Python data analysis and are now part of SciPy Stack 1.0 (http://www.scipy.org/stackspec.html). We will learn how to install SciPy Stack 1.0 and Jupyter Notebook, and write some simple data analysis code as a warm-up exercise.
The following are the libraries available in the Python ecosystem that are used by data analysts and data scientists:
NumPy: This is a general-purpose library that provides numerical arrays, and functions to manipulate the arrays efficiently.
SciPy: This is a scientific computing library that provides science and engineering related functions. SciPy supplements and slightly overlaps NumPy. NumPy and SciPy historically shared their code base but were later separated.
Pandas: This is a data-manipulation library that provides data structures and operations for manipulating tables and time series data.
Matplotlib: This is a 2D plotting library that provides support for producing plots, graphs, and figures. Matplotlib is used by SciPy and supports NumPy.
IPython: This provides a powerful interactive shell for Python, kernel for Jupyter, and support for interactive data visualization. We will cover the IPython shell later in this chapter.
Jupyter Notebook: This provides a web-based interactive shell for creating and sharing documents with live code and visualizations. Jupyter Notebook supports multiple versions of Python through the kernel provided by IPython. We will cover the Jupyter Notebook later in this chapter.
Installation instructions for the other required software will be given throughout the book at the appropriate time. At the end of this chapter, you will find pointers on how to find additional information online if you get stuck or are uncertain about the best way of solving problems:
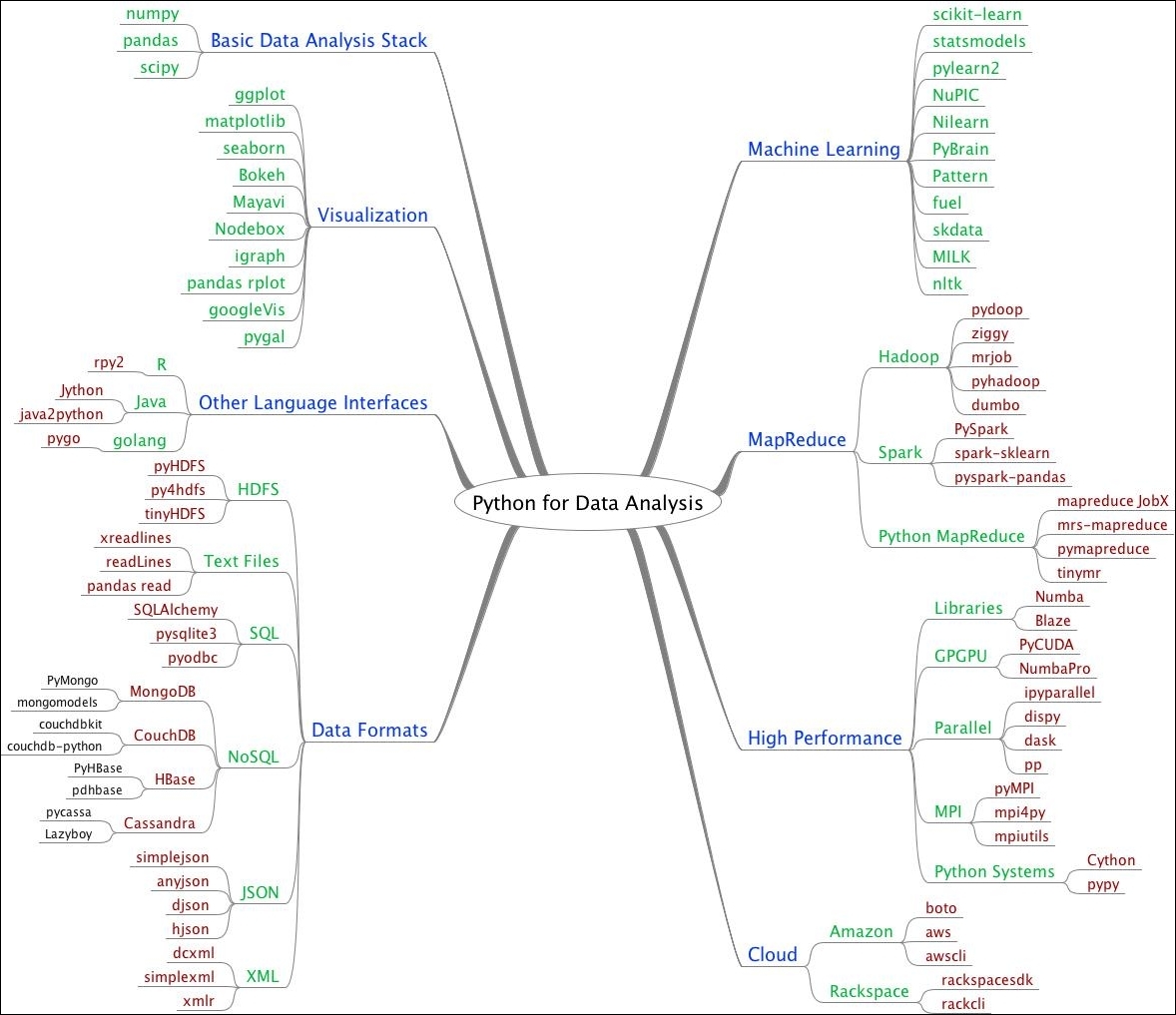
In this chapter, we will cover the following topics:
Installing Python 3
Using IPython as a shell
Reading manual pages
Jupyter Notebook
NumPy arrays
A simple application
Where to find help and references
Listing modules inside the Python libraries
Visualizing data using matplotlib