When we say parametric assumptions, we are referring to base assumptions that algorithms make about the shape of the data. In the previous chapter, while exploring principal component analysis (PCA), we discovered that the end result of the algorithm produced components that we could use to transform data through a single matrix multiplication. The assumption that we were making was that the original data took on a shape that could be decomposed and represented by a single linear transformation (the matrix operation). But what if that is not true? What if PCA is unable to extract useful features from the original dataset? Algorithms such as PCA and linear discriminate analysis (LDA) will always be able to find features, but they may not be useful at all. Moreover, these algorithms rely on a predetermined equation and will always output the same features each and every time they are run. This is why we consider both LDA and PCA as being linear transformations...
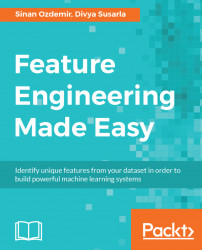
Feature Engineering Made Easy
By :
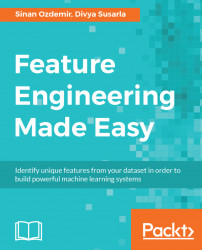
Feature Engineering Made Easy
By:
Overview of this book
Feature engineering is the most important step in creating powerful machine learning systems. This book will take you through the entire feature-engineering journey to make your machine learning much more systematic and effective.
You will start with understanding your data—often the success of your ML models depends on how you leverage different feature types, such as continuous, categorical, and more, You will learn when to include a feature, when to omit it, and why, all by understanding error analysis and the acceptability of your models. You will learn to convert a problem statement into useful new features. You will learn to deliver features driven by business needs as well as mathematical insights. You'll also learn how to use machine learning on your machines, automatically learning amazing features for your data.
By the end of the book, you will become proficient in Feature Selection, Feature Learning, and Feature Optimization.
Table of Contents (14 chapters)
Title Page
Copyright and Credits
Packt Upsell
Contributors
Preface
Introduction to Feature Engineering
Feature Understanding – What's in My Dataset?
Feature Improvement - Cleaning Datasets
Feature Construction
Feature Selection
Feature Transformations
Feature Learning
Case Studies
Other Books You May Enjoy
Customer Reviews