A convolution is a mathematical operation that slides one function over another and measures the integral of their pointwise multiplication. It has deep connections with the Fourier transform and the Laplace transform and is heavily used in signal processing. Convolutional layers actually use cross-correlations, which are very similar to convolutions.
Thus, the most important building block of a CNN is the convolutional layer: neurons in the first convolutional layer are not connected to every single pixel in the input image (like they were in previous chapters), but only to pixels in their receptive fields – see figure 3. In turn, each neuron in the second convolutional layer is connected only to neurons located within a small rectangle in the first layer:
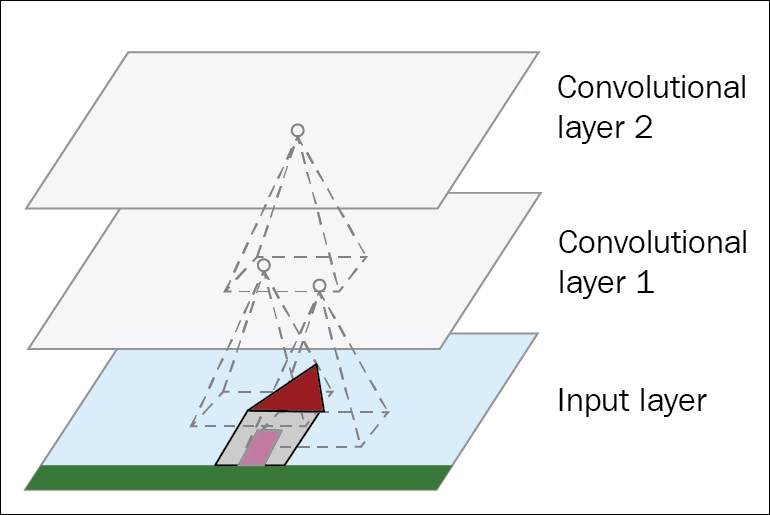
Figure 3: CNN layers with rectangular local receptive fields
This architecture allows the network to concentrate on low-level features in the first hidden layer, then assemble them into higher-level features in the...