The primary biliary cirrhosis data was introduced in first two chapters using the jackknife method. Observations in clinical trials are usually subject to censoring, and the jackknife method helps to complete incomplete observations through the idea of pseudo values. Since pseudo values are likely to be dependent on each other, the generalized estimating equation framework made it possible to estimate the impact of twelve covariates at the time of interest. The idea of pseudo values and the generalized estimating equation framework makes it easy for practitioners to interpret the results. However, this method might not be useful if the number of censored observations is exceptionally high. Furthermore, it is also preferable to have statistical methods that preserve the incompleteness of the observations and yet make good use of them. The general (linear) regression framework with time as the dependent variable and the error term following appropriate...
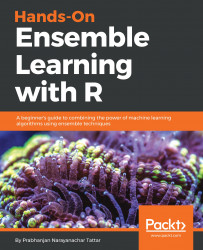
Hands-On Ensemble Learning with R
By :
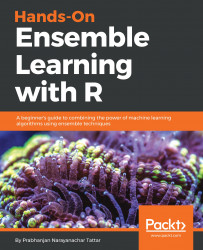
Hands-On Ensemble Learning with R
By:
Overview of this book
Ensemble techniques are used for combining two or more similar or dissimilar machine learning algorithms to create a stronger model. Such a model delivers superior prediction power and can give your datasets a boost in accuracy.
Hands-On Ensemble Learning with R begins with the important statistical resampling methods. You will then walk through the central trilogy of ensemble techniques – bagging, random forest, and boosting – then you'll learn how they can be used to provide greater accuracy on large datasets using popular R packages. You will learn how to combine model predictions using different machine learning algorithms to build ensemble models. In addition to this, you will explore how to improve the performance of your ensemble models.
By the end of this book, you will have learned how machine learning algorithms can be combined to reduce common problems and build simple efficient ensemble models with the help of real-world examples.
Table of Contents (17 chapters)
Hands-On Ensemble Learning with R
Contributors
Preface
Introduction to Ensemble Techniques
Bootstrapping
Bagging
Random Forests
The Bare Bones Boosting Algorithms
Boosting Refinements
The General Ensemble Technique
Ensemble Diagnostics
Ensembling Regression Models
Ensembling Survival Models
Ensembling Time Series Models
What's Next?
Bibliography
Index
Customer Reviews