We're going to be utilizing a new classifier in this chapter, a linear Support Vector Machine (SVM). An SVM is an algorithm that attempts to linearly separate data points into classes using a maximum-margin hyperplane. That's a mouthful, so let's look at what it really means.
Suppose we have two classes of data, and we want to separate them with a line. (We'll just deal with two features, or dimensions, here.) What is the most effective way to place that line? Lets have a look at an illustration:
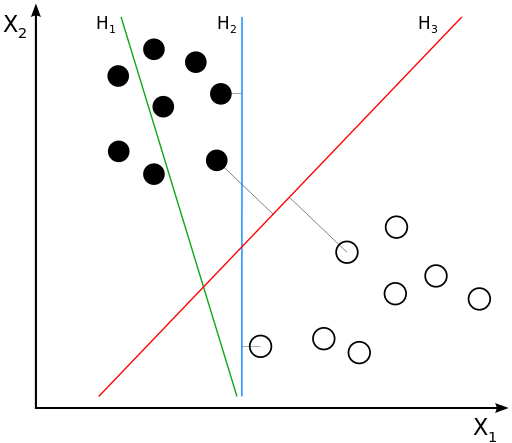
In the preceding diagram, line H1 does not effectively discriminate between the two classes, so we can eliminate that one. Line H2 is able to discriminate between them cleanly, but H3 is the maximum-margin line. This means that the line is centered between the two nearest points of each class, which are known as the support vectors. These...