- No; in a convex set, given two points, the segment connecting them always lies inside the set.
- Considering the radial structure of the dataset, the RBF kernel can generally solve the problem.
- With ε=1.0, many points are not density-reachable. When the radius of the balls is reduced, we should expect more noisy points.
- No; k-medoids can employ any metric.
- No; DBSCAN is not sensitive to the geometry, and can manage any kind of cluster structure.
- We have shown that the performance of mini-batch K-means is slightly worse than k-means. Therefore, the answer is yes. It's possible to save memory by using a batch algorithm.
- Considering that the variance of the noise is σ2=0.005 → σ ≈ 0.07, which is about 14 times smaller than the cluster standard deviation, we cannot expect such a large number of new assignments (80%) in a stable clustering...
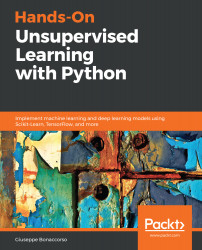
Hands-On Unsupervised Learning with Python
By :
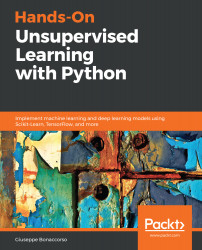
Hands-On Unsupervised Learning with Python
By:
Overview of this book
Unsupervised learning is about making use of raw, untagged data and applying learning algorithms to it to help a machine predict its outcome. With this book, you will explore the concept of unsupervised learning to cluster large sets of data and analyze them repeatedly until the desired outcome is found using Python.
This book starts with the key differences between supervised, unsupervised, and semi-supervised learning. You will be introduced to the best-used libraries and frameworks from the Python ecosystem and address unsupervised learning in both the machine learning and deep learning domains. You will explore various algorithms, techniques that are used to implement unsupervised learning in real-world use cases. You will learn a variety of unsupervised learning approaches, including randomized optimization, clustering, feature selection and transformation, and information theory. You will get hands-on experience with how neural networks can be employed in unsupervised scenarios. You will also explore the steps involved in building and training a GAN in order to process images.
By the end of this book, you will have learned the art of unsupervised learning for different real-world challenges.
Table of Contents (12 chapters)
Preface
Getting Started with Unsupervised Learning
Clustering Fundamentals
Advanced Clustering
Hierarchical Clustering in Action
Soft Clustering and Gaussian Mixture Models
Anomaly Detection
Dimensionality Reduction and Component Analysis
Unsupervised Neural Network Models
Generative Adversarial Networks and SOMs
Assessments
Other Books You May Enjoy
Customer Reviews