Network science can be used to understand systems not just in terms of which parts are connected, but also in terms of how their neighbors and their neighbor's neighbors. In other words, network science can be used to understand the structure of a networked system are connected. The following three chapters describe how network science is used to understand network structure at various scales. This chapter focuses on the small-scale, or micro-scale, structure. The micro-scale structure describes the positions and roles played by specific nodes within the greater network. Such analysis can be used to identify influential individuals, bottlenecks in flows, and convenient locations for assembling information or resources. These properties (and others) can be characterized using centrality measures, which quantify various structural properties...
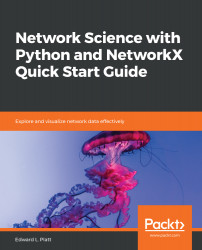
Network Science with Python and NetworkX Quick Start Guide
By :
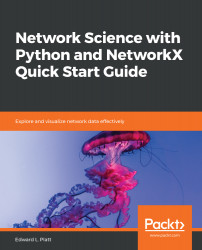
Network Science with Python and NetworkX Quick Start Guide
By:
Overview of this book
NetworkX is a leading free and open source package used for network science with the Python programming language. NetworkX can track properties of individuals and relationships, find communities, analyze resilience, detect key network locations, and perform a wide range of important tasks. With the recent release of version 2, NetworkX has been updated to be more powerful and easy to use.
If you’re a data scientist, engineer, or computational social scientist, this book will guide you in using the Python programming language to gain insights into real-world networks. Starting with the fundamentals, you’ll be introduced to the core concepts of network science, along with examples that use real-world data and Python code. This book will introduce you to theoretical concepts such as scale-free and small-world networks, centrality measures, and agent-based modeling. You’ll also be able to look for scale-free networks in real data and visualize a network using circular, directed, and shell layouts.
By the end of this book, you’ll be able to choose appropriate network representations, use NetworkX to build and characterize networks, and uncover insights while working with real-world systems.
Table of Contents (15 chapters)
Preface
What is a Network?
Working with Networks in NetworkX
From Data to Networks
Affiliation Networks
The Small Scale - Nodes and Centrality
The Big Picture - Describing Networks
In-Between - Communities
Social Networks and Going Viral
Simulation and Analysis
Networks in Space and Time
Visualization
Conclusion
Other Books You May Enjoy
Customer Reviews