In this chapter, we covered topics related to data comparisons. We started by discussing the idea of a data fingerprint. In order to illustrate a data fingerprint, we introduced a hash function that can take any string and convert it to a number in a fixed range. This kind of hash function is useful because it enables us to ensure that data has the right identity – just like a fingerprint in real life. After introducing hash functions, we talked about the need for data signatures. A data signature or analytic signature is useful because it enables us to see whether two datasets are approximate matches – fingerprints require exact matches. We illustrated the use of analytic signatures with image data. We concluded the chapter by covering latent variable models. The latent variable model we discussed was factor analysis. We discussed an application of factor analysis that uses psychological survey data to determine differences between people's personalities. In the next chapter, we...
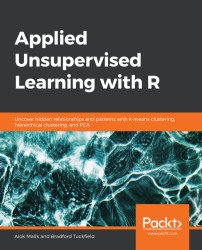
Applied Unsupervised Learning with R
By :
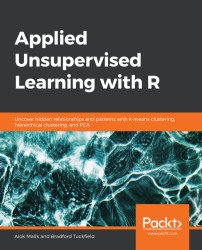
Applied Unsupervised Learning with R
By:
Overview of this book
Starting with the basics, Applied Unsupervised Learning with R explains clustering methods, distribution analysis, data encoders, and features of R that enable you to understand your data better and get answers to your most pressing business questions.
This book begins with the most important and commonly used method for unsupervised learning - clustering - and explains the three main clustering algorithms - k-means, divisive, and agglomerative. Following this, you'll study market basket analysis, kernel density estimation, principal component analysis, and anomaly detection. You'll be introduced to these methods using code written in R, with further instructions on how to work with, edit, and improve R code. To help you gain a practical understanding, the book also features useful tips on applying these methods to real business problems, including market segmentation and fraud detection. By working through interesting activities, you'll explore data encoders and latent variable models.
By the end of this book, you will have a better understanding of different anomaly detection methods, such as outlier detection, Mahalanobis distances, and contextual and collective anomaly detection.
Table of Contents (9 chapters)
Applied Unsupervised Learning with R
Preface
Introduction to Clustering Methods
Advanced Clustering Methods
Probability Distributions
Dimension Reduction
Data Comparison Methods
Anomaly Detection
Customer Reviews