To get the most out of this book
First of all, you'll need Python 3 installed and working on your system. Code examples are tested with spaCy v3.0, however, most of the code is compatible with spaCy v2.3 due to backwards compatibility. For the helper libraries such as scikit-learn, pandas, NumPy, and matplotlib, the latest versions available on pip will work. We use TensorFlow, transformers, and helper libraries starting with Chapter 7, Customizing spaCy Models, so you can install these libraries by the time you reach Chapter 7.
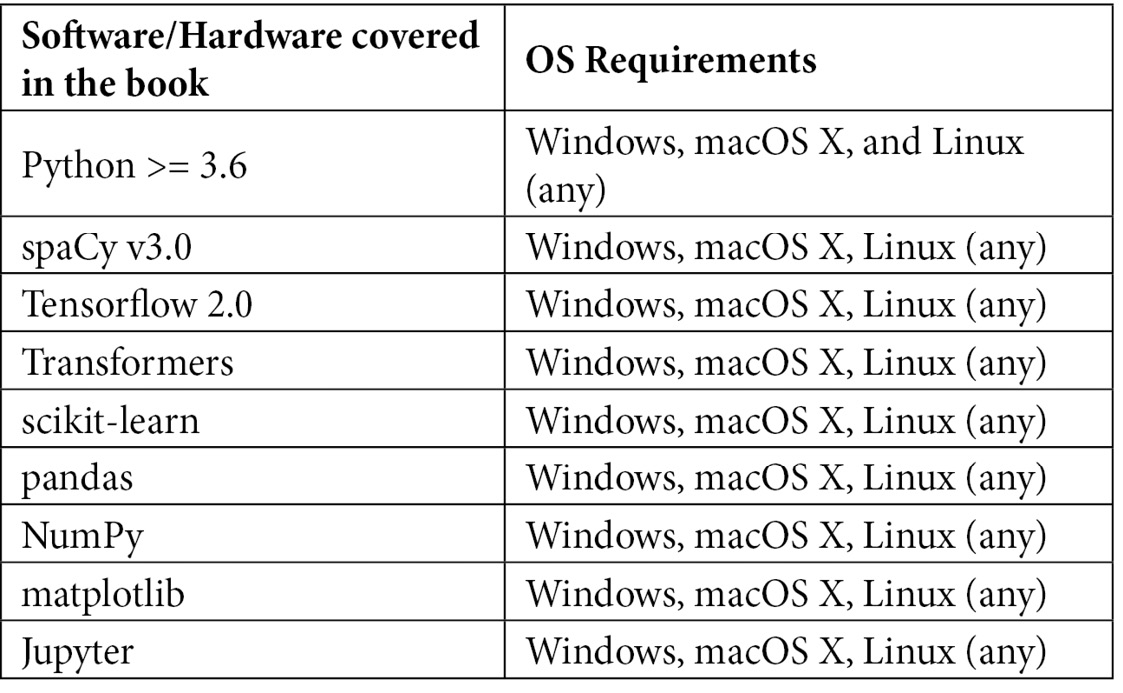
We used Jupyter notebooks from time to time. You can view the notebooks on the book's GitHub page. If you want to work with Jupyter notebooks, that's great; you can install Jupyter via pip. If you don't want to, you can still copy and paste the code into the Python shell and make the code work.
If you are using the digital version of this book, we advise you to type the code yourself or access the code via the GitHub repository (link available in the next section). Doing so will help you avoid any potential errors related to the copying and pasting of code.