Types of ML algorithms
In the last section, we looked at what ML is, and we examined a use case where we had labeled data. In this section, we will look at the four main types of ML approaches to give us a base understanding of what each type does, and where and how they can be applied. The four types of ML algorithms are as follows:
- Supervised learning
- Unsupervised learning
- Semi-supervised learning
- Reinforcement learning
Let’s examine the four types of ML methods, which will serve as a building block for later chapters.
Supervised learning
In supervised learning, an ML model is trained using data made of features and a target. This enables the model to learn the underlying relationship in the data. After training, the model can use its newfound knowledge to make predictions on unseen data. For example, say you want to buy a house. You would consider factors such as the location of the house, the number of rooms, the number of bathrooms, whether it has a garden, and the type of property; these factors you would consider as the features, while the price of the house is the target. Perhaps after the TensorFlow exam, you can roll up your sleeves, scrape some housing data, and train a model to predict house prices based on these features. You can use your house prediction model to compare prices on real estate websites to close a good deal for yourself.
There are two types of supervised learning – regression and classification. In a regression task, the label is a numeric value, just like the example we gave previously in which the target is the predicted price of the house. Conversely, in a classification task, the label is a category, just like the fraud example we discussed previously, in which the goal was to detect whether a transaction was fraudulent or not fraudulent. In a classification task, the model will learn to categorize the target as classes.
When dealing with a classification task made up of two classes (for example, fraudulent and non-fraudulent transactions), it is referred to as binary classification. When there are more than two categories (for example, the classification of different car brands), it is referred to as multi-class classification.
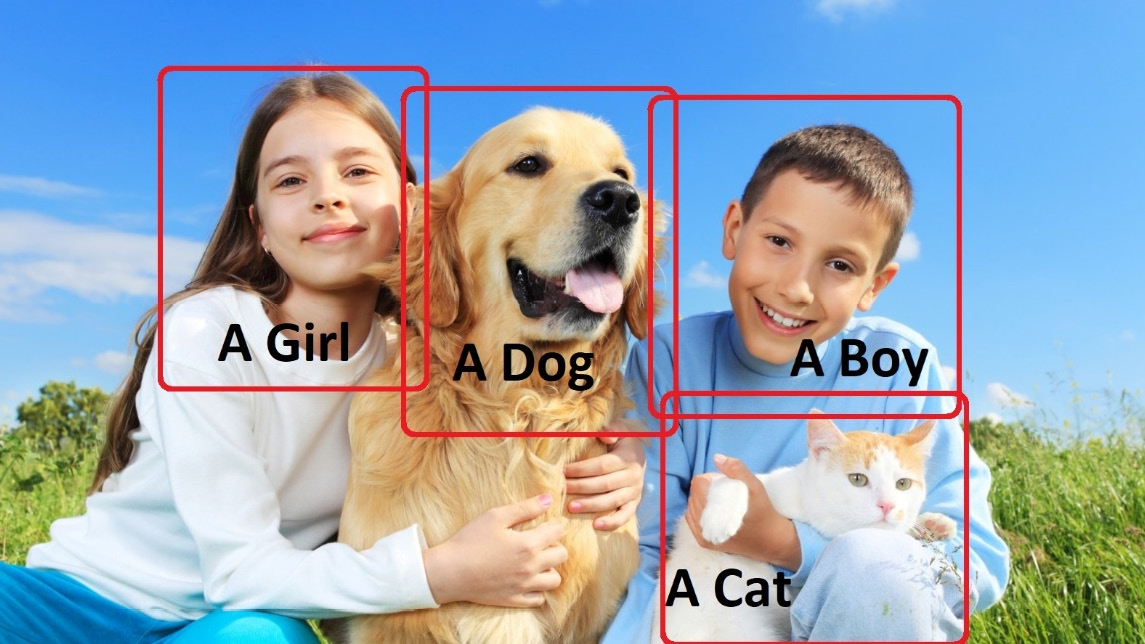
Figure 1.6: An example of multi-label classification, where the model identifies multiple subjects within an image
Multi-label classification is another type of classification, and it is used for image tagging in social media applications such as Facebook and Instagram. Unlike binary and multi-class classification tasks in which we have one target per instance, in multi-label classification our model identifies multiple targets for each instance, as shown in Figure 1.6, where our model identifies a girl, a dog, a boy, and a cat in the given photo.
Unsupervised learning
Unsupervised learning is the opposite of supervised learning. In this case, there is no labeled data. The model will have to figure things out by itself. Here, an unsupervised learning algorithm is provided with data, and we expect it to extract meaningful insights from the unlabeled data, by identifying patterns within the data without relying on predefined targets – for example, an image you work on for a large retail store that aims to segment its customers for marketing purposes. You are given data containing the demographics and spending habits of the store’s customers. By employing an unsupervised ML model, you successfully cluster customers with similar characteristics into distinct customer segments. The marketing team can now create a tailored campaign targeted at each of the customer segments identified by the model, potentially leading to a higher conversion rate from the campaign.
Semi-supervised learning
Semi-supervised learning is a combination of supervised and unsupervised learning. In this case, there is some data with labels (i.e., it has both features and a target) and the rest is without labels. In such scenarios, the unlabeled data is usually the dominant group. In this case, we can apply a combination of unsupervised and supervised learning methods to generate optimal results, especially when the cost implication and time required to manually label the data may not be practical. Here, the model takes advantage of the available label data to learn the underlying relationships, which it then applies to the unlabeled data.
Imagine you work for a big corporation that collects a large volume of documents that we need to classify and send to the appropriate department (i.e, finance, marketing, and sales) for effective document management, and only a small number of the documents are labeled. Here, we apply semi-supervised learning, train the model on the labeled document, and apply the learned patterns to classify the remaining unlabeled documents.
Reinforcement Learning
In reinforcement learning, unlike supervised learning, the model does not learn from training data. Instead, it learns from its interactions within an environment; it gets rewarded for making the right decisions and punished for making wrong choices. It is a trial-and-error learning approach in which the model learns from its past experience to make better decisions in the future. The model aims to maximize reward. Reinforcement learning is applied in self-driving cars, robotics, trading and finance, question answering, and text summarization, among other exciting use cases.
Now that we can clearly differentiate between the different types of ML approaches, we can look into what the core components of an ML life cycle are and what steps we should take, from the birth of a project to its application by end users. Let us take a look at the ML life cycle.