What is data sourcing?
Data sourcing refers to connecting your pipeline environment to all data input sources relevant to your data pipeline. Many applications require the use of structured data, as well as unstructured and semi-structured data, to make effective and timely decisions. This is depicted in the following diagram:
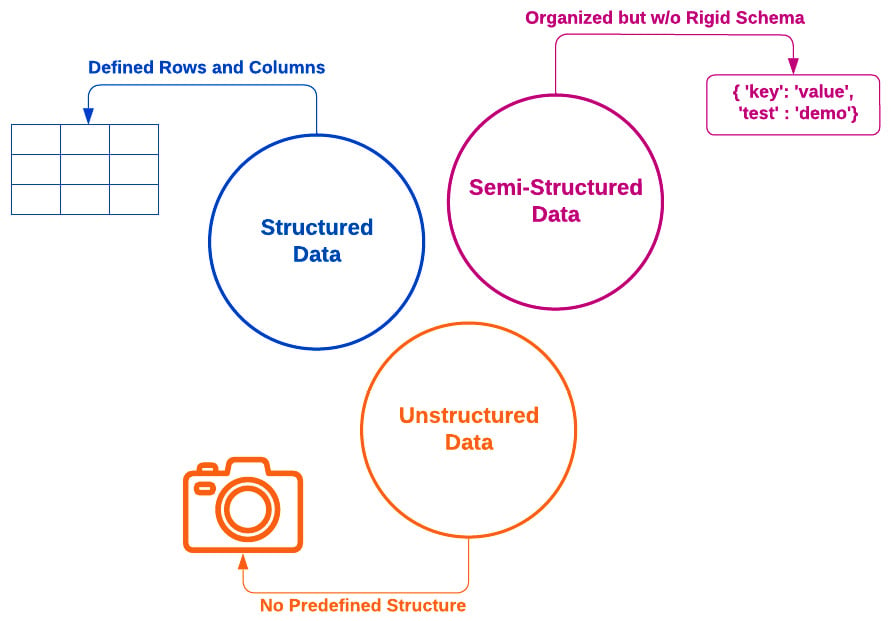
Figure 4.1 – Structured, semi-structured, and unstructured data definitions
All this data can be acquired from two types of sources: internal and external, where internal data refers to sources related to a company’s business operations and external data refers to any data sourced outside of an organization (see Reference #1 in the References section). While these terms are relative to the specific context of the organization and may or may not come into play for your own data pipeline projects, integrating both internal and external data helps produce an insightful, incredibly powerful output data product that can...