Chapter 2
Minimal Machine Learning Solution
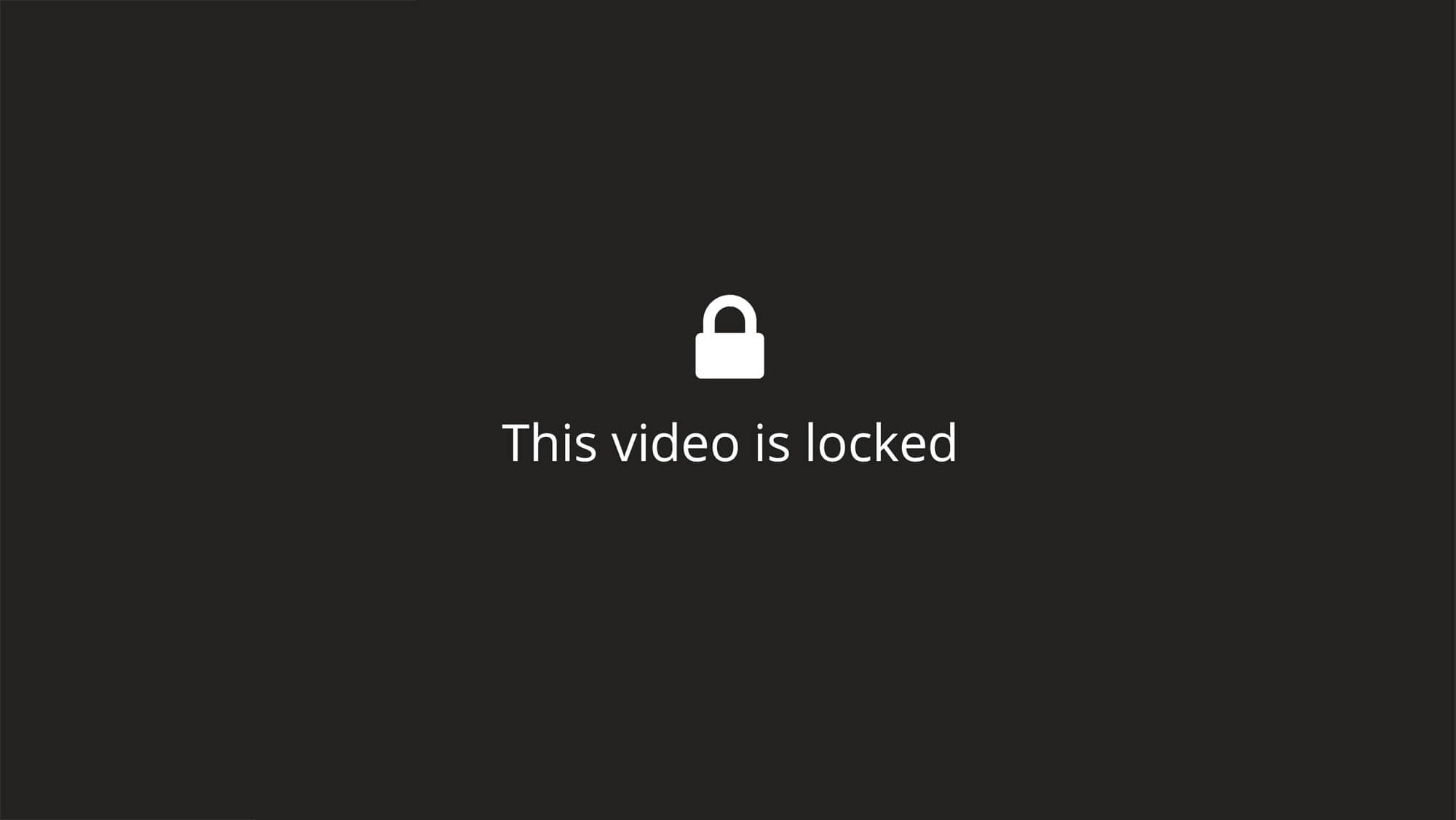
Section 2
Cross-Validation Using Various Algorithms
Iris dataset has only 150 observations. To make the most out of the set, we will employ cross-validation. Also, we wanted to compare the performance of two different classifiers, support vector classifier and logistic regression. Cross-validation will help us with this comparison issue as well. - Import and load the iris dataset - Create an instance of an SVC classifier and fit it - Predict and examine the SVC and logistic regression's performance on X_test_2