As mentioned previously, the partitioner and replication scheme is motivated by the Dynamo paper; let's talk about it in detail.
Cassandra is a peer-to-peer system with no single point of failure; the cluster topology information is communicated via the Gossip protocol. The Gossip protocol is similar to real-world gossip, where a node (say B) tells a few of its peers in the cluster what it knows about the state of a node (say A). Those nodes tell a few other nodes about A, and over a period of time, all the nodes know about A.
The key feature of Cassandra is the ability to scale incrementally. This includes the ability to dynamically partition the data over a set of nodes in the cluster. Cassandra partitions data across the cluster using consistent hashing and randomly distributes the rows over the network using the hash of the row key. When a node joins the ring, it is assigned a token that advocates where the node has to be placed in the ring:
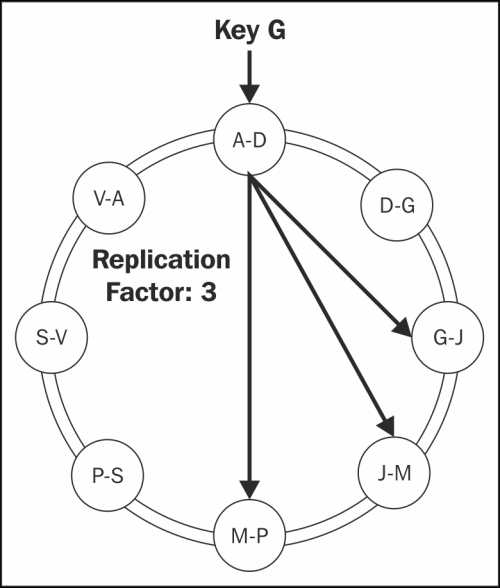
Now consider a case where the replication factor is 3; clients randomly write or read from a coordinator (every node in the system can act as a coordinator and a data node) in the cluster. The node calculates a hash of the row key and provides the coordinator enough information to write to the right node in the ring. The coordinator also looks at the replication factor and writes to the neighboring nodes in the ring order. More on vnodes and multi-DC clusters will be discussed in later chapters.
Given a sufficient period of time over which no changes are sent, all updates can be expected to propagate through the system and the replicas created will be consistent. Cassandra supports both the eventual consistency model and strong consistency model, which can be controlled from the client while performing an operation.
Cassandra supports various consistency levels while writing or reading data. The consistency level drives the number data replicas the coordinator has to contact to get the data before acknowledging the clients. If W + R > Replication Factor, where W is the number of nodes to block on write and R the number to block on reads, the clients will see a strong consistency behavior:
ONE: R/W at least one node
TWO: R/W at least two nodes
QUORUM: R/W from at least floor (N/2) + 1, where N is the replication factor
When nodes are down for maintenance, Cassandra will store hints for updates performed on that node, which can be delivered back when the node is available in the future. To make data consistent, Cassandra relies on hinted handoffs, read repairs, and anti-entropy repairs. We will talk about them in detail in later chapters.