Machine learning tasks or machine learning processes are typically classified into three broad categories, depending on the nature of the learning feedback available to a learning system. Supervised learning, unsupervised learning, and reinforcement learning; these three kinds of machine learning tasks are shown in Figure 3, and will be discussed in this section:
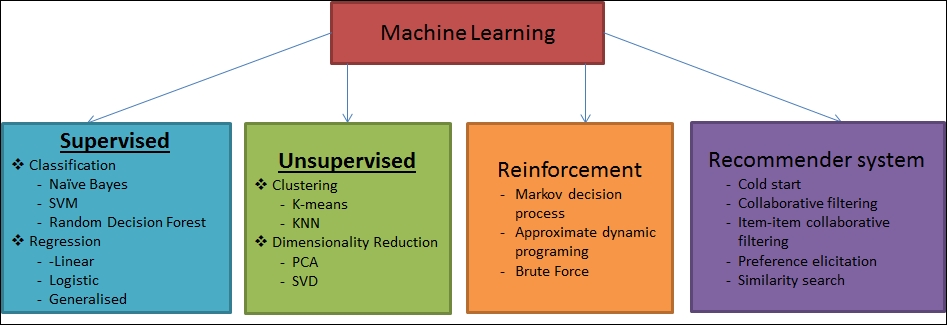
Figure 3: Machine learning tasks.
A supervised learning application makes predictions based on a set of examples, and the goal is to learn general rules that map inputs to outputs aligning with the real world. For example, a dataset for spam filtering usually contains spam messages as well as non-spam messages. Therefore, we could know which messages in a training set are spams or non-spams. Nevertheless, we might have the opportunity to use this information to train our model in order to classify new and unseen messages. Figure 4 shows the schematic diagram of the supervised learning.
In other...