In the previous sections, we leveraged plotting capability from the arules
and igraph
packages to plot induced rules. In this section, we introduce arulesViz
, a package dedicated to plot association rules, generated by the arules
package. The arulesViz
package integrates seamlessly with the arules
packages in terms of sharing data structures.
The following code is quite self-explanatory. A multitude of graphs, including interactive/non-interactive scatter plots, graph plots, matrix plots, and group plots can be generated from the rules data structure; it's a great visual way to explore the rules induced:
######################################################################## # # R Data Analysis Projects # # Chapter 1 # # Building Recommender System # A step step approach to build Association Rule Mining # # Script: # # RScript to explore arulesViz package # for Association rules visualization # # Gopi Subramanian #########################################################################
library(arules) library(arulesViz)
get.txn <- function(data.path, columns){ # Get transaction object for a given data file # # Args: # data.path: data file name location # columns: transaction id and item id columns. # # Returns: # transaction object transactions.obj <- read.transactions(file = data.path, format = "single", sep = ",", cols = columns, rm.duplicates = FALSE, quote = "", skip = 0, encoding = "unknown") return(transactions.obj) }
get.rules <- function(support, confidence, transactions){ # Get Apriori rules for given support and confidence values # # Args: # support: support parameter # confidence: confidence parameter # # Returns: # rules object parameters = list( support = support, confidence = confidence, minlen = 2, # Minimal number of items per item set maxlen = 10, # Maximal number of items per item set target = "rules" ) rules <- apriori(transactions, parameter = parameters) return(rules)
support <- 0.01 confidence <- 0.2
# Create transactions object columns <- c("order_id", "product_id") ## columns of interest in data file data.path = '../../data/data.csv' ## Path to data file transactions.obj <- get.txn(data.path, columns) ## create txn object
# Induce Rules all.rules <- get.rules(support, confidence, transactions.obj)
# Scatter plot of rules plotly_arules(all.rules, method = "scatterplot", measure = c("support","lift"), shading = "order")
# Interactive scatter plots plot(all.rules, method = NULL, measure = "support", shading = "lift", interactive = TRUE)
# Get top rules by lift sub.rules <- head(sort(all.rules, by="lift"), 15
# Group plot of rules plot(sub.rules, method="grouped")
# Graph plot of rule plot(sub.rules, method="graph", measure = "lift")
The following diagram is the scatter plot of rules induced:
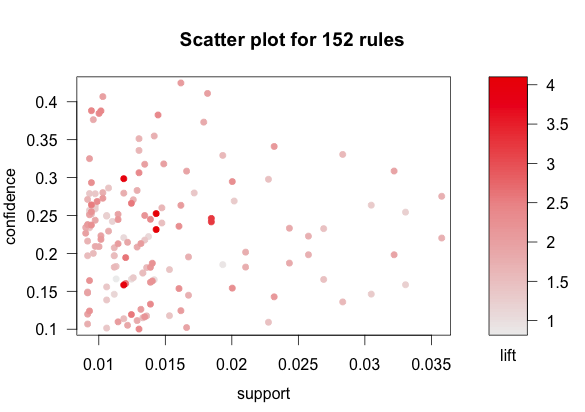
The following diagram is the grouped plot of rules induced:
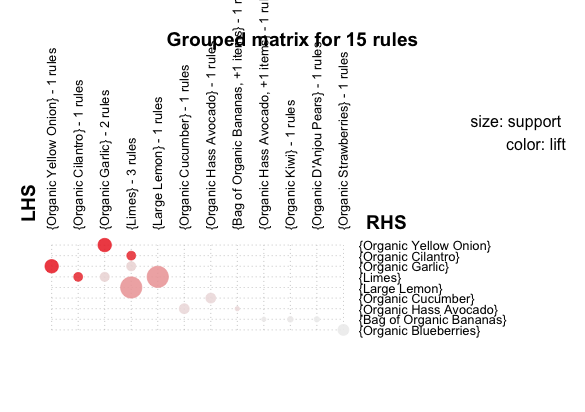
The following diagram is the graph plot of rules induced:
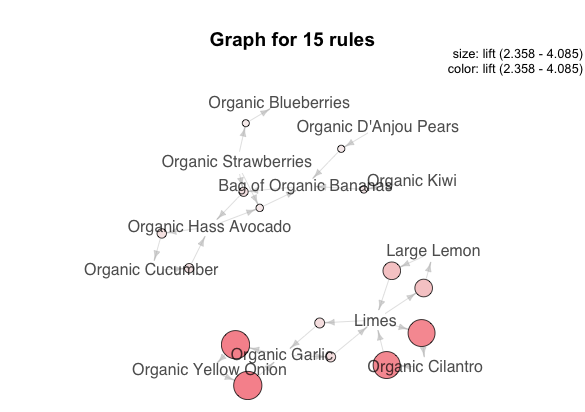