Let's consider building an e-mail spam filter using machine learning. Here we are interested in two classes: spam for unsolicited messages and non-spam for regular e-mails:
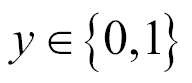
The first challenge is that given an e-mail, how do we represent it as feature vector x. An e-mail is just a bunch of text or a collection of words (therefore, this problem domain falls into a broader category called text classification). Let's represent an e-mail with a feature vector with the length equal to the size of the dictionary. If a given word in a dictionary appears in an e-mail, the value will be 1, otherwise 0. Let's build a vector representing the e-mail with the online pharmacy sale content:
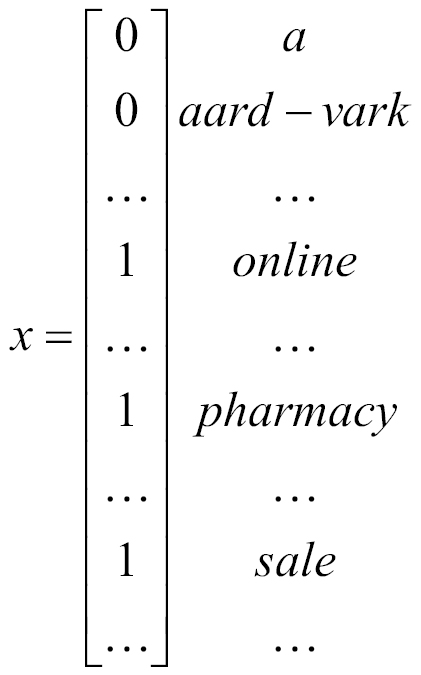
The dictionary of words in this feature vector is called vocabulary, and the dimensions of the vector are the same as the size of the vocabulary. If the vocabulary size is 10,000, the possible values in this feature vector will be 210,000.
Our goal is to model the probability of x...