Now let's understand a common partner to pooling layers. In this section, we're going to learn about max pooling layers being similar to convolutional layers, although they have some differences in common usage. We'll wrap up by showing how these layers can be combined for maximum effect.
Suppose you've used a convolutional layer to extract a feature from an image and suppose hypothetically, you had a small weight matrix that detects a dog shape in the window of the image.
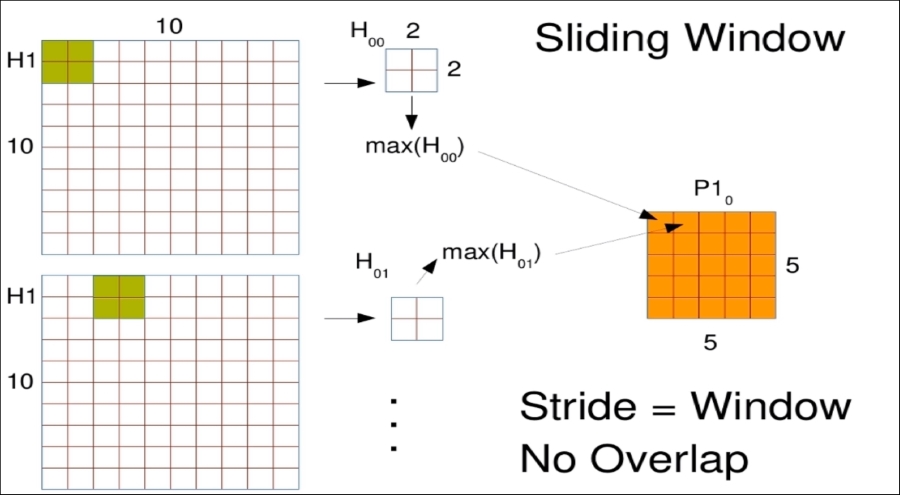
When you convolve this around your output is likely to report many nearby regions with dog shapes. But this is really just due to the overlap. There probably aren't many dogs all next to each other, though maybe an image of puppies would. You'd really only like to see that feature once and preferably wherever it is strongest. The max pooling layer attempts to do this. Like a convolutional layer a pooling layer works on a small sliding windows of an image.
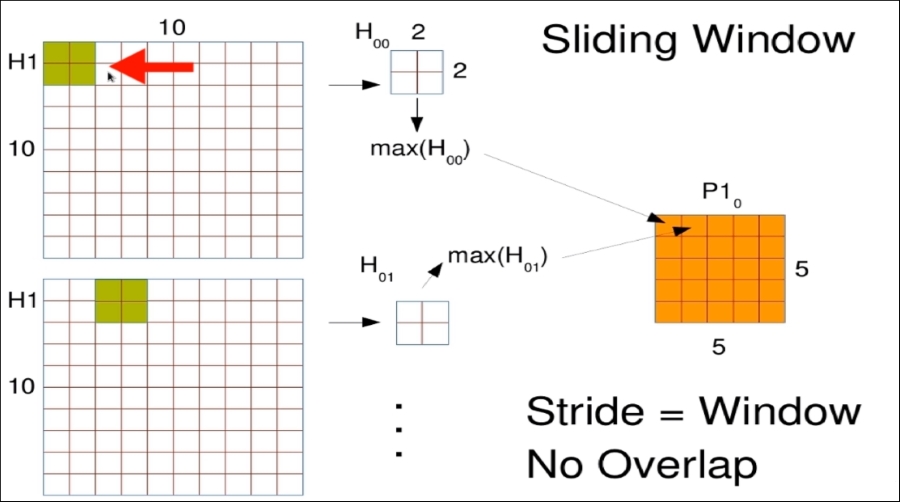
Typically, researchers add...