We've seen the surprising way in which the key structure of a CQL table maps to the underlying column family representation, but so far the values stored in CQL have mapped completely transparently into cell values at the column family level. While this is true for scalar data types, something much more interesting happens where collections are concerned.
We'll start by looking at sets, which are the simplest of the three collection column types. Let's take a look at alice
's row in the user_status_updates
column family:
GET user_status_updates['alice'];
Recall that, by only using the bracket operator once in the GET
statement, we'll retrieve all cells in the alice
RowKey.
There are quite a few cells in alice
's wide row, but we're particularly interested in those having to do with the starred_by_users
column, which is a set:

Note that the cell names here look like the cell names in home_status_updates
, but with yet another component: a short hexadecimal string appended to the end. Unfortunately, there's no straightforward way to get cassandra-cli to print those hexadecimal strings in a human-readable form, so you'll have to take my word for it that 0x626f62
is the blob encoding of the string bob
, and 0x6361726f6c
encodes the string carol
.
With this cleared up, we can observe that the underlying representation of a CQL set is a collection of cells, with each cell encoding one of the items in the set. The item is actually encoded in the cell's name by appending it to the usual combination of clustering column value and row name that comprises the name of a scalar cell.
Let's have a look at a set using the visual comparison we have been developing in the course of this chapter. For clarity, I've omitted everything unrelated to the set:
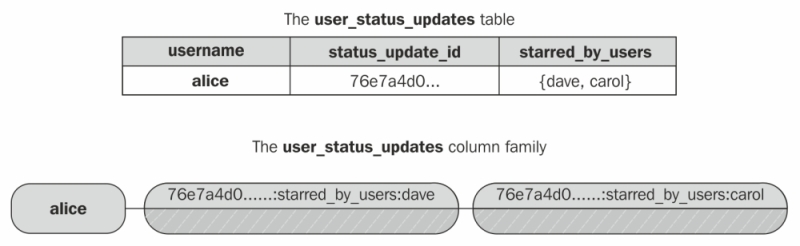
This turns out to be a very effective way to represent a set at the column family level. When a new item is added to a set, the CQL abstraction simply adds a cell containing the item appended to the appropriate clustering column/column name combination. Removing items from the set is as simple as deleting the cell that encodes that item. In both cases, Cassandra can do the right thing without having to read the existing contents of the set.
Although maps might seem like the most complex of the three collection column types, their column family level representation is actually simpler than that of lists, so we'll save lists for last. To get a sense of what map columns look like at the column family level, let's take a look at the social_identities map in alice
's user record:
GET users['alice'];
Again, we'll focus solely on the interesting cells in the result:

The structure of the cell names looks identical to the cell names used by set columns. However, unlike set columns, the cells encoding map columns have values in them. In a map, the map keys are stored in cell names, and the map values are stored in cell values. We can thus visualize a map like this:
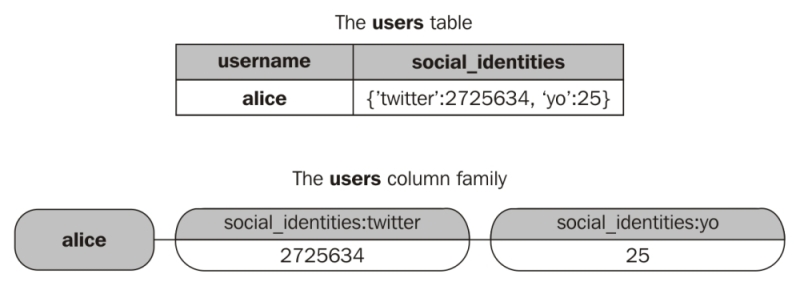
The relationship between the underlying representations of map columns and set columns should come as no great surprise; many programming languages implement their set data type using an underlying map whose values are not meaningful.
Our reasoning about the process of insertion and deletion in sets also carries over to maps. Since, for any given map key that needs to be inserted or deleted, the CQL abstraction can calculate the corresponding cell name, map key-value pairs can be directly inserted and deleted without Cassandra needing to read the full contents of the column.
The last collection type we'll explore at the column family level is lists. Lists are the most complex collection types in a couple of ways. As you learned in Chapter 8, Collections, Tuples, and User-defined Types, lists have more available mutation operations than maps or sets. It also turns out that lists have the most complex underlying representation.
Let's take a look at the shared_by
list in the user_status_updates
table. Before we dive into the underlying structure, let's quickly open a cqlsh console and add a new value to the shared_by
list of alice
. When we last left this list, it only had a single username in it, which isn't really useful for the purposes of our exploration:
UPDATE "user_status_updates" SET "shared_by" = "shared_by" + ['bob'], "shared_by" = ['dave'] + "shared_by" WHERE "username" = 'alice' AND "id" = 76e7a4d0-e796-11e3-90ce-5f98e903bf02;
Note that, by setting the shared_by
column twice in our UPDATE
statement, we can both prepend and append values to the list in a single query. Having done this, let's take a look at alice
's partition in the user_status_updates
column family, focusing on the cells related to the shared_by
collection:

At first glance, the structure looks quite similar to that of set and map columns: the cell name consists of a clustering column value, a data column name, and finally some other list-related component. However, what is the list-related component?
It turns out that this value is a UUID; the hex string printed in cassandra-cli is in fact the usual UUID representation, just without the dashes. If we decode the UUID e358efe0678711e4ac53f51fa8d64e2e
, the last one in the list, we'll find that it encodes the timestamp at which we appended bob
to the list column.
Our observation allows us to deduce that Cassandra appends items to list columns by creating a new cell whose name includes the current timestamp UUID. Since the value of timestamp UUIDs is monotonically increasing, this will ensure that the appended value is the last in the list, since it has the highest (newest) UUID. This way, Cassandra can construct the cell name for a newly appended list item without consulting the current contents of the list.
Now, let's take a look at the first cell in the list, the one whose value contains dave
. Recall that we prepended dave
onto the list, so we know that the cell name doesn't contain the current timestamp because that would put Dave at the end of the list, not the beginning.
Decoding the UUID in Dave's cell's name, 77d9443f854711d9ac53f51fa8d64e2e
, yields a timestamp several years old—February 23, 2005. Where does this timestamp come from? As it turns out, the following calculation yields it:
Calculate how much time has elapsed since midnight UTC on January 1, 2010
Calculate the timestamp corresponding to that duration before January 1, 2010
So while the UUIDs used to append to lists are monotonically increasing, the UUIDs used to prepend are monotonically decreasing, since we'll be subtracting an ever-greater duration from the "epoch" time of January 1, 2010. Since the first version of Cassandra to support lists was released in early 2013, we can assume that that particular epoch marker was chosen to ensure that all generated prepend timestamps would be smaller than all generated append timestamps.
We've seen that the structure of maps and sets allows Cassandra to perform all supported mutation operations on those collection types without having to read the currently stored values. Unfortunately, the same is not true for lists. While appending and prepending to lists can be done discretely, other operations require scanning the existing contents of the list first.
If we take a moment to ponder the underlying representation of list columns, the reason is clear. If, for instance, we ask to remove the value dave
from the list, there's no way for Cassandra to know which cell(s) contain that value without reading it. Even in the simpler case of deleting an element at a given position, Cassandra must look at the cell names to know which cell is at the position requested. So, it's worth keeping in mind that list mutations—except for appending and prepending—are naturally less performant than mutations on other collection types.