In this chapter, we will use the power of SQL queries to address non-linear datasets. Creating datasources in Redshift or RDS gives us the potential for upstream SQL-based feature engineering prior to the datasource creation. We implemented a similar approach in Chapter 4, Loading and Preparing the Dataset, by leveraging the new AWS Athena service to apply preliminary transformations on the data before creating the datasource. This enabled us to expand the Titanic
dataset by creating new features, such as the Deck
number, replacing the Fare
with its log or replacing missing values for the Age
variable. The SQL transformations were simple, but allowed us to expand the original dataset in a very flexible way. The AWS Athena service is S3 based. It allows us to run SQL queries on datasets hosted on S3 and dump the results in S3 buckets. We were still creating Amazon ML datasources from S3, but simply adding an extra data preprocessing layer to massage...
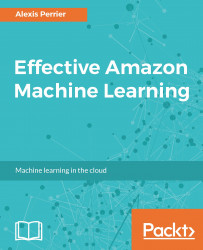
Effective Amazon Machine Learning
By :
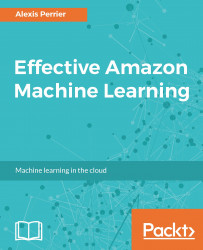
Effective Amazon Machine Learning
By:
Overview of this book
Predictive analytics is a complex domain requiring coding skills, an understanding of the mathematical concepts underpinning machine learning algorithms, and the ability to create compelling data visualizations. Following AWS simplifying Machine learning, this book will help you bring predictive analytics projects to fruition in three easy steps: data preparation, model tuning, and model selection.
This book will introduce you to the Amazon Machine Learning platform and will implement core data science concepts such as classification, regression, regularization, overfitting, model selection, and evaluation. Furthermore, you will learn to leverage the Amazon Web Service (AWS) ecosystem for extended access to data sources, implement realtime predictions, and run Amazon Machine Learning projects via the command line and the Python SDK.
Towards the end of the book, you will also learn how to apply these services to other problems, such as text mining, and to more complex datasets.
Table of Contents (17 chapters)
Title Page
Credits
About the Author
About the Reviewer
www.PacktPub.com
Customer Feedback
Dedication
Preface
Introduction to Machine Learning and Predictive Analytics
Machine Learning Definitions and Concepts
Overview of an Amazon Machine Learning Workflow
Loading and Preparing the Dataset
Model Creation
Predictions and Performances
Command Line and SDK
Creating Datasources from Redshift
Building a Streaming Data Analysis Pipeline
Customer Reviews