The .NET Framework has been around for general release since 2002. The base of the framework is the Common Language Runtime or CLR. The CLR is a virtual machine that abstracts much of the OS specific functionality like memory management and exception handling. The CLR is loosely based on the Java Virtual Machine (JVM). Sitting on top of the CLR is the Framework Class Library (FCL) that allows different languages to interoperate with the CLR and each other: the FCL is what allows VB.Net, C#, F#, and Iron Python code to work side-by-side with each other.
Since its first release, the .NET Framework has included more and more features. The first release saw support for the major platform libraries like WinForms, ASP.NET, and ADO.NET. Subsequent releases brought in things like Windows Communication Foundation (WCF), Language Integrated Query (LINQ), and Task Parallel Library (TPL). At the time of writing, the latest version is of the .Net Framework is 4.6.2.
In addition to the full-Monty .NET Framework, over the years Microsoft has released slimmed down versions of the .NET Framework intended to run on machines that have limited hardware and OS support. The most famous of these releases was the Portable Class Library (PCL) that targeted Windows RT applications running Windows 8. The most recent incantation of this is Universal Windows Applications (UWA), targeting Windows 10.
At Connect()
; in November 2015, Microsoft announced GA of the latest edition of the .NET Framework. This release introduced the .Net Core 5. In January, they decided to rename it to .Net Core 1.0. .NET Core 1.0 is intended to be a slimmed down version of the full .NET Framework that runs on multiple operating systems (specifically targeting OS X and Linux). The next release of ASP.NET (ASP.NET Core 1.0) sits on top of .NET Core 1.0. ASP.NET Core 1.0 applications that run on Windows can still run the full .NET Framework.
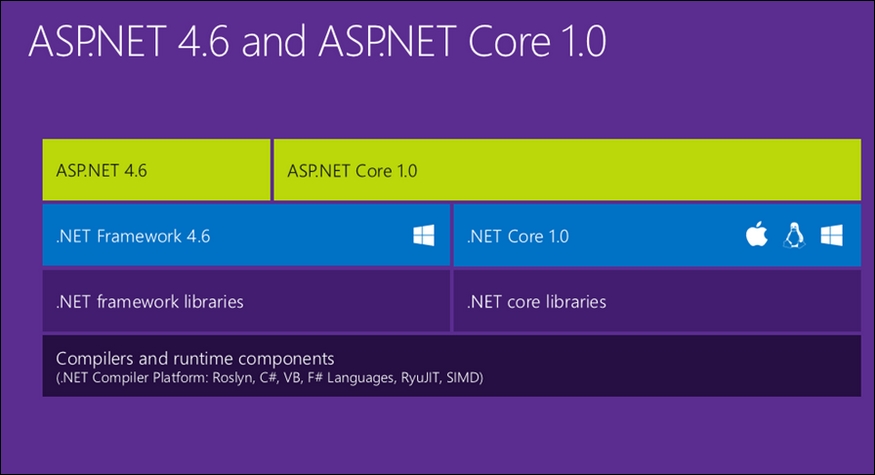
In this book, we will be using a mixture of ASP.NET 4.0, ASP.NET 5.0, and Universal Windows Applications. As you can guess, machine learning models (and the theory behind the models) change with a lot less frequency than framework releases so the most of the code you write on .NET 4.6 will work equally well with PCL and .NET Core 1.0. Saying that, the external libraries that we will use need some time to catch up—so they might work with PCL but not with .NET Core 1.0 yet. To make things realistic, the demonstration projects will use .NET 4.6 on ASP.NET 4.x for existing (Brownfield) applications. New (Greenfield) applications will be a mixture of a UWA using PCL and ASP.NET 5.0 applications.