Throughout the chapters in this book, we have seen various machine learning models, each progressively increasing their perceptual abilities. By this, I mean that we were first introduced to a model capable of classifying a single object present in an image. Then came a model that was able to classify not only multiple objects but also their corresponding bounding boxes. In this chapter, we continue this progression by introducing semantic segmentation, in other words, being able to assign each pixel to a specific class, as shown in the following figure:
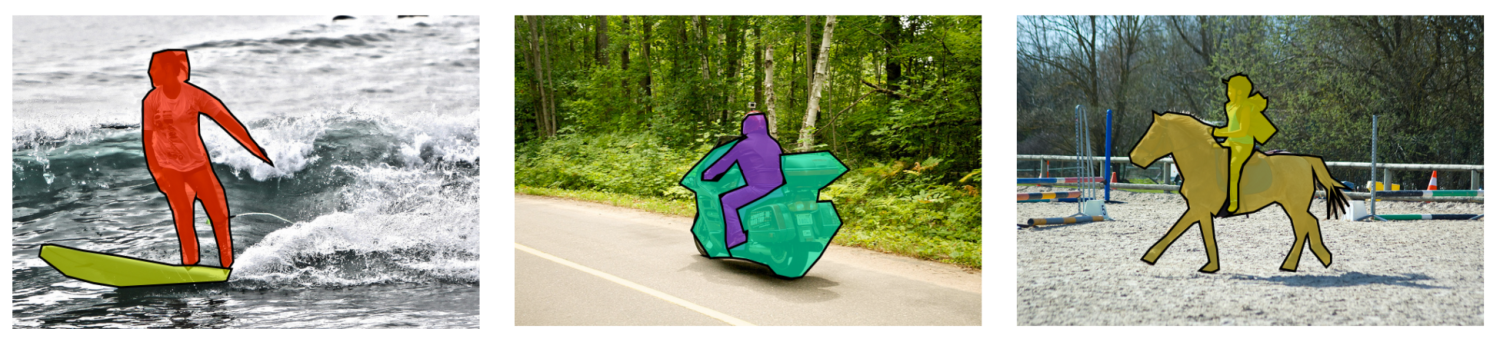
Source: http://cocodataset.org/#explore
This allows for a greater understanding of the scene and, therefore, opportunities for more intelligible interfaces and services. But this is not the main focus of this chapter. In this chapter, we will use semantic segmentation to create an image effects application as a way to demonstrate imperfect predictions. We'll be using this to motivate a discussion...