This book introduces data mining with popular free Python libraries. It is written in a conversational style, aiming to be approachable while imparting intuition on the reader. Data mining is a broad field of analytical methods designed to uncover insights from your data that are not obvious or discoverable by conventional analysis techniques. The field of data mining is vast, so the topics in this quick start guide were chosen by their relevance to not only their field of origin, but also the adjacent applications of machine learning and artificial intelligence. After a procedural first half, focused on getting the reader comfortable with data collection, loading, and munging, the book will move to a completely conceptual discussion. The concepts are introduced from first principles intuition and broadly grouped as transformation, clustering, and prediction. Popular methods such as principal component analysis, k-means clustering, support vector machines, and random forest are all covered in the conceptual second half of the book. The book ends with a discussion on pipe-lining and deploying your analytical models.
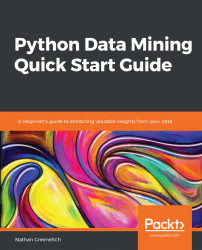
Python Data Mining Quick Start Guide
By :
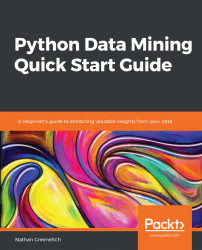
Python Data Mining Quick Start Guide
By:
Overview of this book
Data mining is a necessary and predictable response to the dawn of the information age. It is typically defined as the pattern and/ or trend discovery phase in the data mining pipeline, and Python is a popular tool for performing these tasks as it offers a wide variety of tools for data mining.
This book will serve as a quick introduction to the concept of data mining and putting it to practical use with the help of popular Python packages and libraries. You will get a hands-on demonstration of working with different real-world datasets and extracting useful insights from them using popular Python libraries such as NumPy, pandas, scikit-learn, and matplotlib. You will then learn the different stages of data mining such as data loading, cleaning, analysis, and visualization. You will also get a full conceptual description of popular data transformation, clustering, and classification techniques.
By the end of this book, you will be able to build an efficient data mining pipeline using Python without any hassle.
Table of Contents (9 chapters)
Preface
Data Mining and Getting Started with Python Tools
Basic Terminology and Our End-to-End Example
Collecting, Exploring, and Visualizing Data
Cleaning and Readying Data for Analysis
Grouping and Clustering Data
Prediction with Regression and Classification
Advanced Topics - Building a Data Processing Pipeline and Deploying It
Other Books You May Enjoy
Customer Reviews