High-Performance Computing Fundamentals
High-Performance Computing (HPC) impacts every aspect of your life, from your morning coffee to driving a car to get to the office, knowing the weather forecast, your vaccinations, movies that you watch, flights that you take, games that you play, and many other aspects. Many of our actions leave a digital footprint, leading to the generation of massive amounts of data. In order to process such data, we need a large amount of processing power. HPC, also known as accelerated computing, aggregates the computing power from a cluster of nodes and divides the work among various interconnected processors, to achieve much higher performance than could be achieved by using a single computer or machine, as shown in Figure 1.1. This helps in solving complex scientific and engineering problems, in critical business applications such as drug discovery, flight simulations, supply chain optimization, financial risk analysis, and so on:
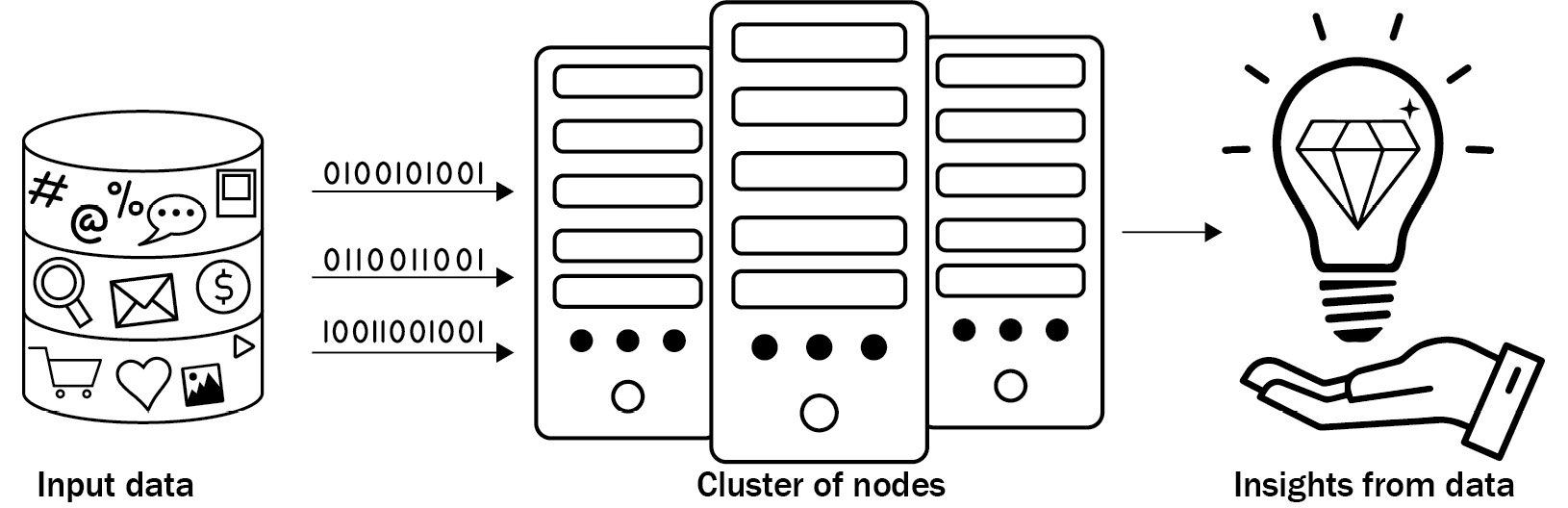
Figure 1.1 – HPC
For example, drug discovery is a data-intensive process, which involves computationally heavy calculations to simulate how virus protein binds with human protein. This is an extremely expensive process and may take weeks or months to finish. With the unification of Machine Learning (ML) with accelerated computing, researchers can simulate drug interactions with protein with higher speed and accuracy. This leads to faster experimentation and significantly reduces the time to market.
In this chapter, we will learn the fundamentals and importance of HPC, followed by technological advancements in the area. We will understand the constraints and how developers can benefit from the elasticity of the cloud while still optimizing costs to innovate faster to gain a competitive business advantage.
In this chapter, we will cover the following topics:
- Why do we need HPC?
- Limitations of on-premises HPC
- Benefits of doing HPC on the cloud
- Driving innovation across industries with HPC