Join our book community on Discord
https://packt.link/EarlyAccessCommunity
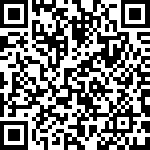
In this chapter, we’ll introduce working with time series data in the Python programming language. We will cover how to install Python and libraries in Python. This chapter will help you set up everything you need to get started with the book.
Python has become one of the most popular languages for data science. It is a powerful, easy-to-read, general-purpose high-level programming language. Additionally, the Python community has built a large number of useful packages for data analysis, which can be easily installed using the pip package manager
The most popular Python packages for data science are collected in the so-called SciPy stack, which includes packages such as NumPy, pandas, scikit-learn, and matplotlib.
In this chapter, we’ll start by installing Python and lots of libraries. Then we will introduce pandas, NumPy, and matplotlib, and a few other libraries and modules that will come in...