In Chapter 9, Multithreading with Distributed Computing, we looked at running the same task across a number of compute nodes in a cluster system. The main goal of such a setup is to process data in a highly parallel fashion, theoretically speeding up said processing relative to a single system with fewer CPU cores.
GPGPU (General Purpose Computing on Graphics Processing Units) is in some ways similar to this, but with one major difference: while a compute cluster with only regular CPUs is good at scalar tasks--meaning performing one task on one single set of data (SISD)--GPUs are vector processors that excel at SIMD (Single Input, Multiple Data) tasks.
Essentially, this means that one can send a large dataset to a GPU, along with a single task description, and the GPU will proceed to execute that same task on parts of that data in parallel on its hundreds or thousands of cores. One can thus regard a GPU as a very specialized kind of cluster:
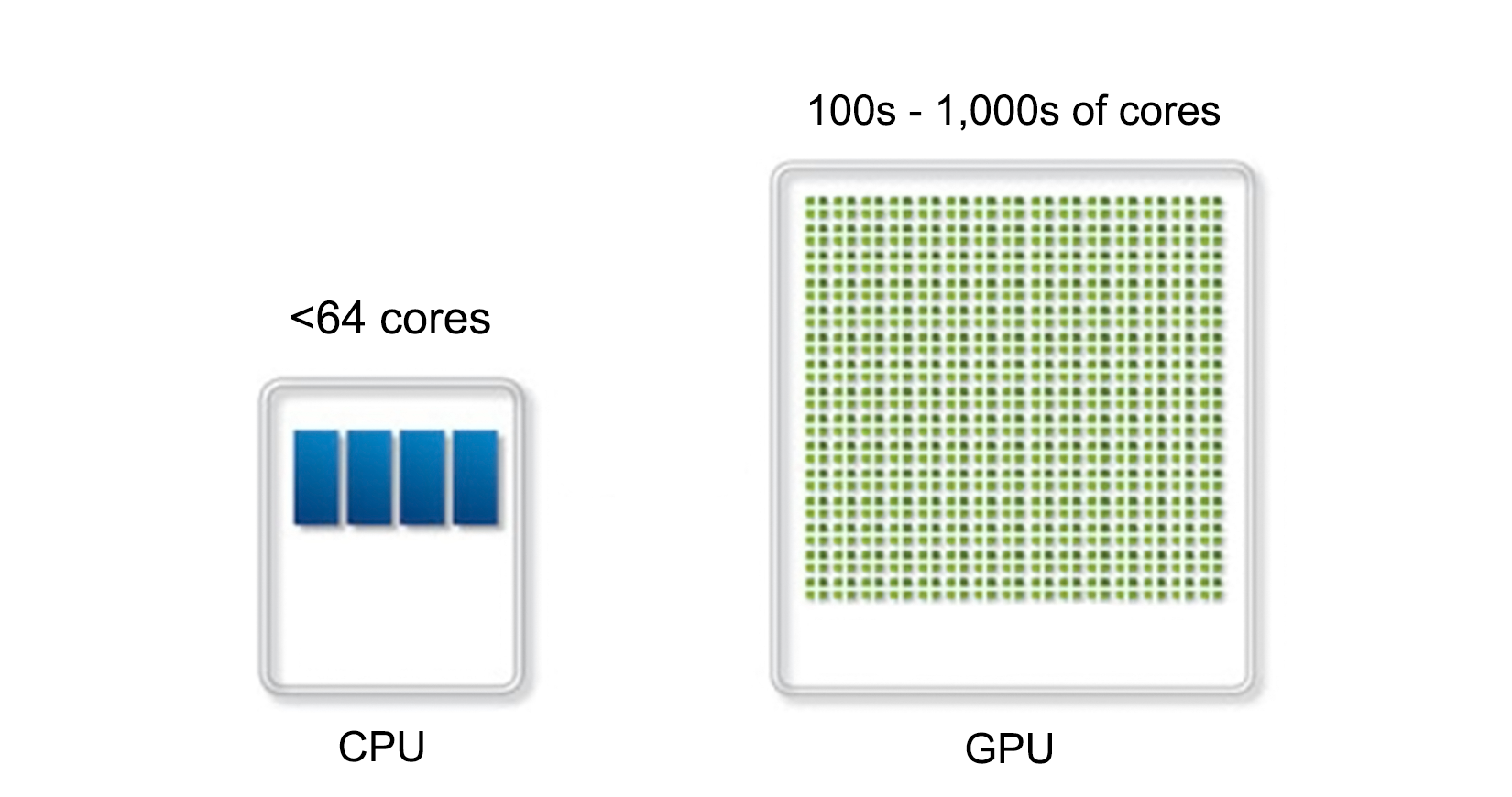