Today banking software landscape scenario deals with many enterprise applications that play an important role in automating banking processes. Each of these applications talk with each other over wire. A typical enterprise architecture landscape consists of software for core banking application, CMS, credit card management, B2B portal, treasury management, HRMS, ERP, CRM, business warehouse, accounting, BI tools, analytics, custom applications, and various other enterprise applications fused together to ensure smooth business processes. The data center for such a complex landscape is usually placed across the globe in different countries with high performance servers having backup and replication. It, in turn, brings in a completely diversified set of software together in a secured environment.
Most of the banks today offer web-based interactions; they not only automate their own business processes, but also access various third party software of other banks and vendors. There is a dedicated team of administrators working 24 x 7 over monitoring and handling of issues/failures and escalations. A simple application of transferring money from your savings bank account to a loan account may touch upon at least twenty different applications. These systems generate terabytes of data everyday, which include transactional data, change logs, and so on.
The problem arises when any business workflow/transaction fails. With such a complex system, it becomes a big task for system administrators/managers to:
Identify the error(s)/issue(s)
Look for errors at different log files, and find out the real problem
Find out the cause of failure
Find correlation among the failures
Monitor the workflow for occurrence of same issue
Product-based software provides nice user interface for administration, monitoring, and log management. However, most of the software including custom built applications and packaged software do not provide any such way. Eventually, the administrators have to get down to operating system file level and start looking for logs.
This is the classic case where Big Data search (Apache Hadoop with Apache Solr) over a distributed environment can be used for effectively monitoring these applications. A sample user interface satisfying some of the expectations is shown in the following screenshot:
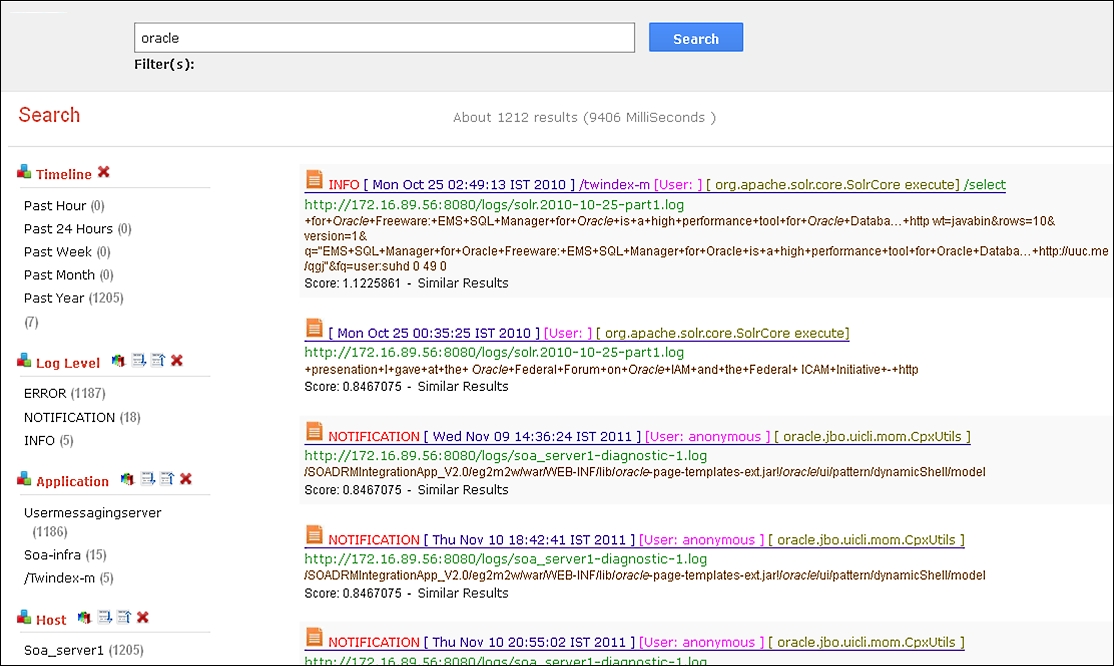
The following reasons enable us to qualify Apache Solr-based Big Data search as the solution:
Apache Hadoop provides an environment for distributed storage and computing for banking global landscape. It also makes your log management scalable in terms of organization growth. This means even if the logs are lost due to rotational log management system from applications or cleaned automatically by your application server, they remain available in a distributed environment of Hadoop.
Apache Solr supports storage of any type of schema making it work with different types of applications having different model layer, that is, application specific log files with their own proprietary schema for each application.
Apache Solr provides efficient searching capabilities with highlighted text and snippets of matched results; this can be suitable while looking for the right set of issues and their occurrences in the past.
Apache Solr provides rich browsing experience in terms of faceted search to drill down to the correct set of results one is looking for. In this case, administrators will be blessed with different types of facets such as timeline-based, application-scoped, based on error types, and severity.
Apache Solr's near real-time search capabilities add value in terms of monitoring and hunt for new logs. One can develop custom utilities that can alert the administrator in case he/she receives a log with high severity. Overall the system gets proactive instead of being reactive.
The overall cost of building this system is less, as none of these technologies require high-end servers, and they are open source.
The overall design, as shown in the following diagram, can have a schema containing common attributes across all the log files such as date and time of log, severity, application name, user name, type of log, and so on. Other attributes can be added as dynamic text fields.
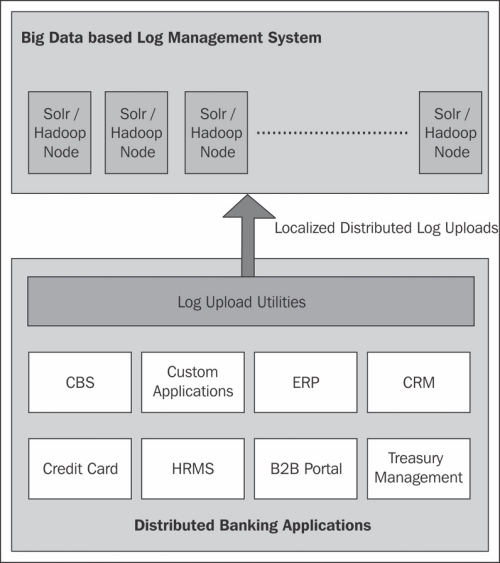
Since each system has different log schema, these logs have to be parsed periodically, and then uploaded to the distributed search. For that, we can either write down the utilities which will understand the schema, and extract the field data from logs. These utilities can feed the outcome to distributed search nodes which are nothing but the Solr instances running on a distributed system like Hadoop. To achieve near real-time search, the Solr configuration requires a change accordingly.