Very high dimensional inputs, such as images or videos, put immense stress on the memory, computation, and operational requirements of traditional machine learning models. In Chapter 3 , Convolutional Neural Network, we have shown how replacing the matrix multiplication by discrete convolutional operations with small kernel resolves these problems. Going forward, Desjardins and Bengio [123] have shown that this approach also works fine when applied to RBMs. In this section, we will discuss the functionalities of this model.
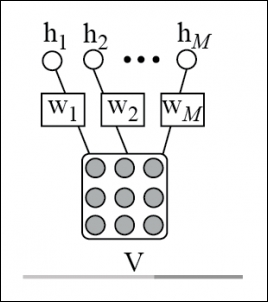
Figure 5.7 : Figure shows the observed variables or the visible units of an RBM can be associated with mini batches of image to a compute the final result. The weight connections represents a set of filters
Further, in normal RBMs, the visible units are directly related to all the hidden variables through different parameters and weights. To describe an image in terms of spatially local features ideally needs fewer parameters...