As an example, if we know that our friend Mary feels cold when it is 10 degrees Celsius, but warm when it is 25 degrees Celsius, then in a room where it is 22 degrees Celsius, the nearest neighbor algorithm would guess that our friend would feel warm, because 22 is closer to 25 than to 10.
Suppose we would like to know when Mary feels warm and when she feels cold, as in the previous example, but in addition, wind speed data is also available when Mary was asked if she felt warm or cold:
Temperature in degrees Celsius |
Wind speed in km/h |
Mary's perception |
10 |
0 |
Cold |
25 |
0 |
Warm |
15 |
5 |
Cold |
20 |
3 |
Warm |
18 |
7 |
Cold |
20 |
10 |
Cold |
22 |
5 |
Warm |
24 |
6 |
Warm |
We could represent the data in a graph, as follows:
Now, suppose we would like to find out how Mary feels at the temperature 16 degrees Celsius with a wind speed of 3km/h using the 1-NN algorithm:
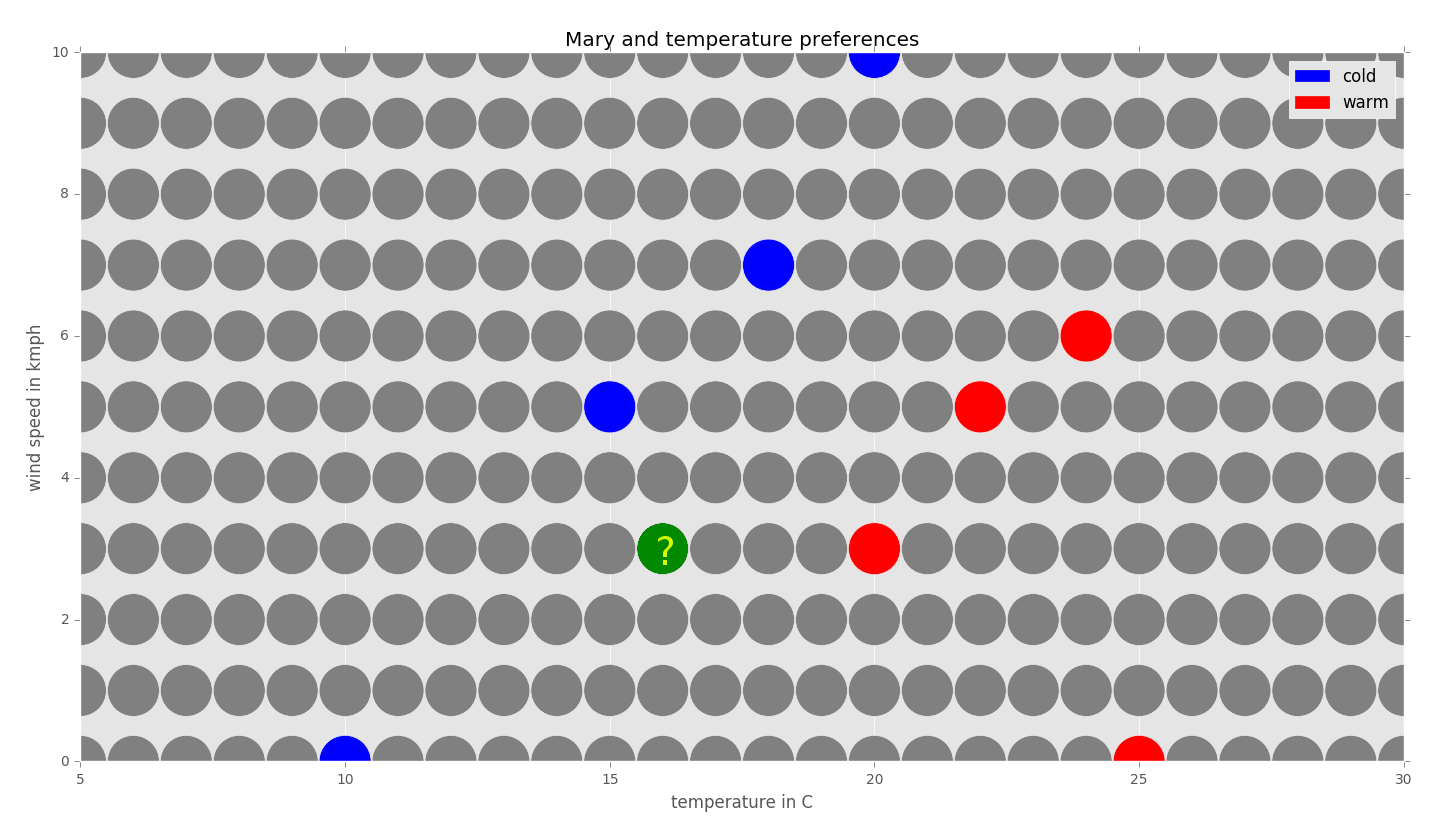
For simplicity, we will use a Manhattan metric to measure the distance between the neighbors on the grid. The Manhattan distance dMan of a neighbor N1=(x1,y1) from the neighbor N2=(x2,y2) is defined to be dMan=|x1- x2|+|y1- y2|.
Let us label the grid with distances around the neighbors to see which neighbor with a known class is closest to the point we would like to classify:
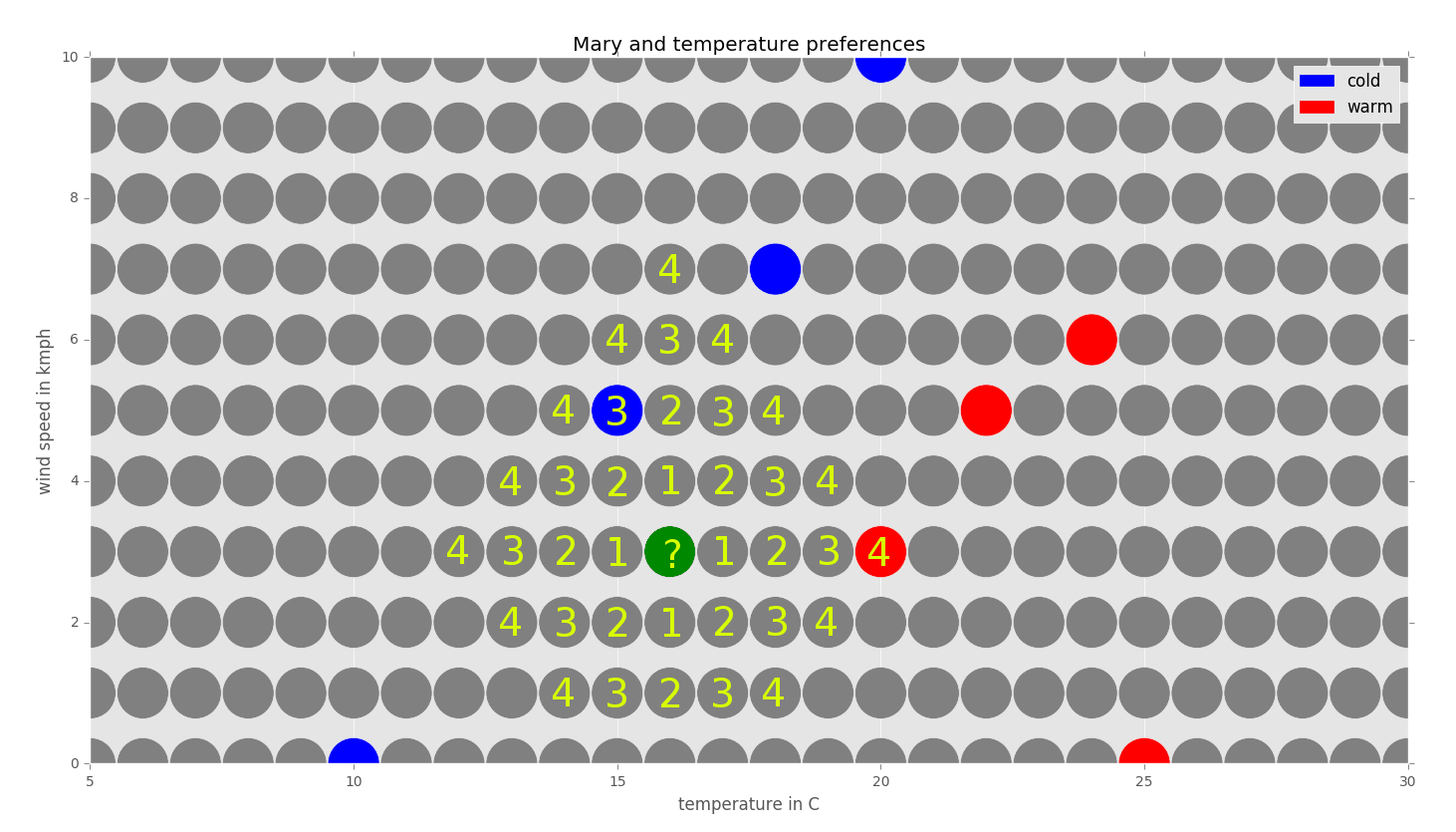
We can see that the closest neighbor with a known class is the one with the temperature 15 (blue) degrees Celsius and the wind speed 5km/h. Its distance from the questioned point is three units. Its class is blue (cold). The closest red (warm) neighbor is distanced four units from the questioned point. Since we are using the 1-nearest neighbor algorithm, we just look at the closest neighbor and, therefore, the class of the questioned point should be blue (cold).
By applying this procedure to every data point, we can complete the graph as follows:
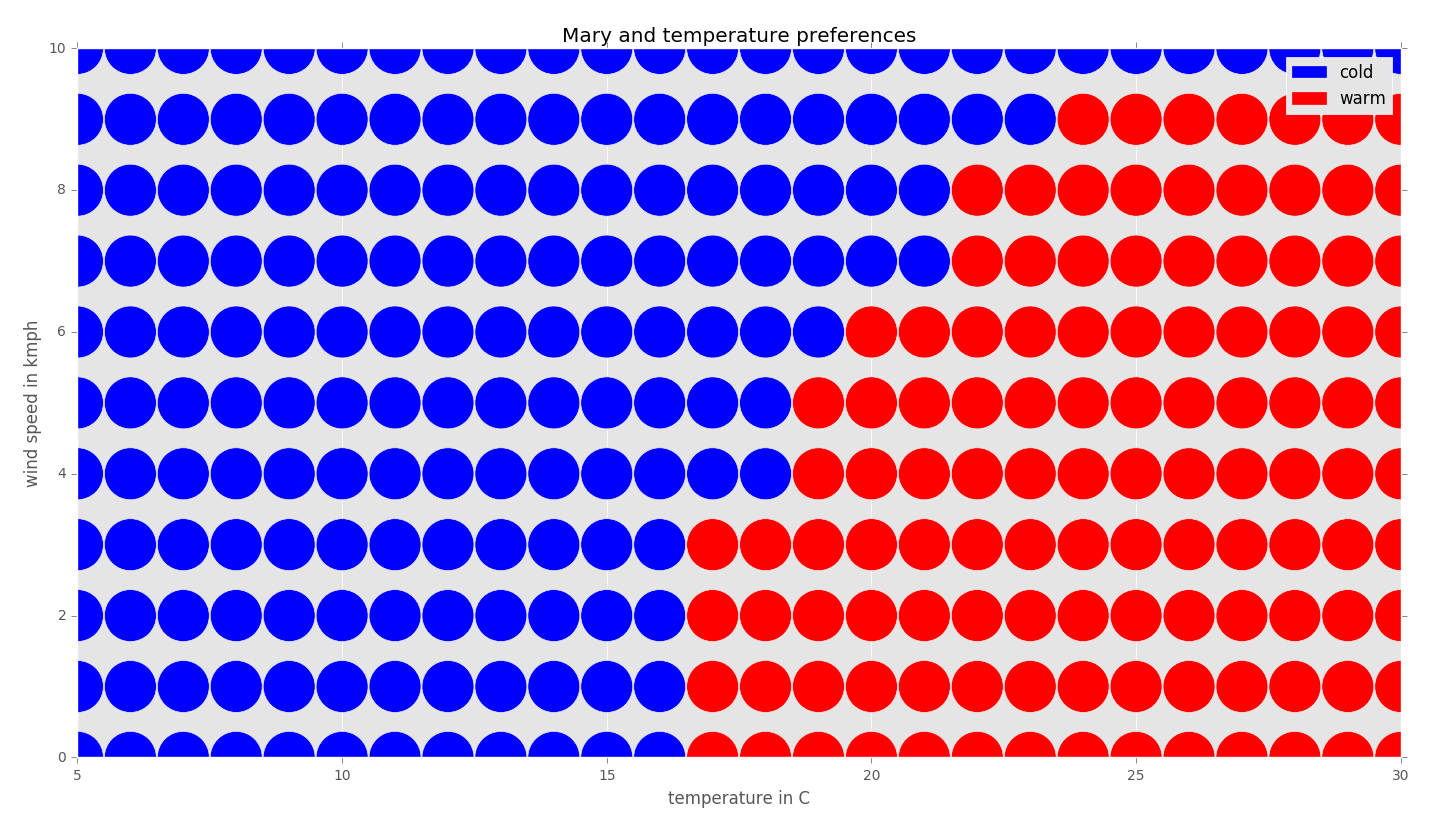
Note that sometimes a data point can be distanced from two known classes with the same distance: for example, 20 degrees Celsius and 6km/h. In such situations, we could prefer one class over the other or ignore these boundary cases. The actual result depends on the specific implementation of an algorithm.