The summary of the logistic regression model produced with the glm()
function has a similar format to that of the linear regression model produced with the lm()
function. This shows us that, for our categorical variables, we have one fewer binary features than the number of levels in the original variable, so for example, the three-valued THAL
input feature produced two binary variables labeled THAL6
and THAL7
. We'll begin by looking first at the regression coefficients that are predicted with our model. These are presented with their corresponding z-statistic. This is analogous to the t-statistic that we saw in linear regression, and again, the higher the absolute value of the z-statistic, the more likely it is that this particular feature is significantly related to our output variable. The p-values next to the z-statistic express this notion as a probability and are annotated with stars and dots, as they were in linear regression, indicating the smallest...
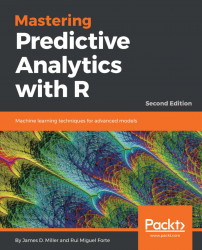
Mastering Predictive Analytics with R - Second Edition
By :
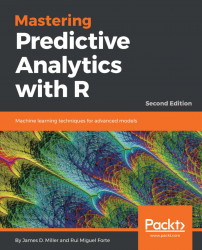
Mastering Predictive Analytics with R - Second Edition
By:
Overview of this book
R offers a free and open source environment that is perfect for both learning and deploying predictive modeling solutions. With its constantly growing community and plethora of packages, R offers the functionality to deal with a truly vast array of problems.
The book begins with a dedicated chapter on the language of models and the predictive modeling process. You will understand the learning curve and the process of tidying data. Each subsequent chapter tackles a particular type of model, such as neural networks, and focuses on the three important questions of how the model works, how to use R to train it, and how to measure and assess its performance using real-world datasets. How do you train models that can handle really large datasets? This book will also show you just that. Finally, you will tackle the really important topic of deep learning by implementing applications on word embedding and recurrent neural networks.
By the end of this book, you will have explored and tested the most popular modeling techniques in use on real- world datasets and mastered a diverse range of techniques in predictive analytics using R.
Table of Contents (22 chapters)
Mastering Predictive Analytics with R Second Edition
Credits
About the Authors
About the Reviewer
www.PacktPub.com
Customer Feedback
Preface
Gearing Up for Predictive Modeling
Tidying Data and Measuring Performance
Linear Regression
Generalized Linear Models
Neural Networks
Support Vector Machines
Tree-Based Methods
Dimensionality Reduction
Ensemble Methods
Probabilistic Graphical Models
Topic Modeling
Recommendation Systems
Scaling Up
Index
Customer Reviews