Regularization is another way to modify the role of variables in a regression model to prevent overfitting and to achieve simpler functional forms. The interesting aspect of this alternative approach is that it actually doesn't require manipulating your original dataset, making it suitable for systems that learn and predict online from large amounts of features and observations, without human intervention. Regularization works by enriching the learning process using a penalization for too complex models to shrink (or reduce to zero) coefficients relative to variables that are irrelevant for your prediction term or are redundant, as they are highly correlated with others present in the model (the collinearity problem seen before).
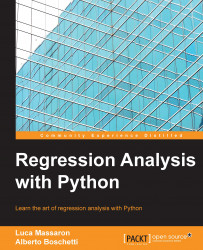
Regression Analysis with Python
By :
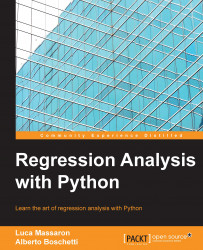
Regression Analysis with Python
By:
Overview of this book
Regression is the process of learning relationships between inputs and continuous outputs from example data, which enables predictions for novel inputs. There are many kinds of regression algorithms, and the aim of this book is to explain which is the right one to use for each set of problems and how to prepare real-world data for it. With this book you will learn to define a simple regression problem and evaluate its performance. The book will help you understand how to properly parse a dataset, clean it, and create an output matrix optimally built for regression. You will begin with a simple regression algorithm to solve some data science problems and then progress to more complex algorithms. The book will enable you to use regression models to predict outcomes and take critical business decisions. Through the book, you will gain knowledge to use Python for building fast better linear models and to apply the results in Python or in any computer language you prefer.
Table of Contents (16 chapters)
Regression Analysis with Python
Credits
About the Authors
About the Reviewers
www.PacktPub.com
Preface
Regression – The Workhorse of Data Science
Approaching Simple Linear Regression
Multiple Regression in Action
Logistic Regression
Data Preparation
Achieving Generalization
Online and Batch Learning
Advanced Regression Methods
Real-world Applications for Regression Models
Index
Customer Reviews