Anyone who is interested in machine learning (ML) would have certainly heard that 80% of a data scientist or machine learning engineer's time is spent on preparing the data, and the remaining 20% is spent on building and evaluating the model. The considerable time spent preparing the data is considered as an investment to construct a good model. A simple model this is made using an excellent dataset outpaces a complex model developed using a lousy dataset. In real life, finding a reliable dataset is very difficult. We have to create and nurture good data. You must be wondering, how do you create good data? This is something that we will discover in this chapter. We will study everything that is needed to create an excellent and viable dataset. In theory, good is relative to what task we have at hand and how we perceive and consume the data. In this chapter...
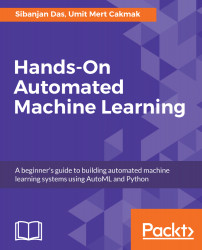
Hands-On Automated Machine Learning
By :
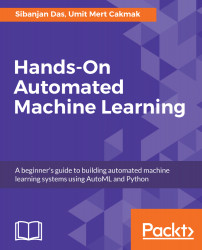
Hands-On Automated Machine Learning
By:
Overview of this book
AutoML is designed to automate parts of Machine Learning. Readily available AutoML tools are making data science practitioners’ work easy and are received well in the advanced analytics community. Automated Machine Learning covers the necessary foundation needed to create automated machine learning modules and helps you get up to speed with them in the most practical way possible.
In this book, you’ll learn how to automate different tasks in the machine learning pipeline such as data preprocessing, feature selection, model training, model optimization, and much more. In addition to this, it demonstrates how you can use the available automation libraries, such as auto-sklearn and MLBox, and create and extend your own custom AutoML components for Machine Learning.
By the end of this book, you will have a clearer understanding of the different aspects of automated Machine Learning, and you’ll be able to incorporate automation tasks using practical datasets. You can leverage your learning from this book to implement Machine Learning in your projects and get a step closer to winning various machine learning competitions.
Table of Contents (10 chapters)
Preface
Introduction to AutoML
Introduction to Machine Learning Using Python
Data Preprocessing
Automated Algorithm Selection
Hyperparameter Optimization
Creating AutoML Pipelines
Dive into Deep Learning
Critical Aspects of ML and Data Science Projects
Other Books You May Enjoy
Customer Reviews