Approaching CNNs
As outlined in Chapter 5, Introducing Artificial Neural Networks Modeling, ANNs draw their inspiration from biological NNs. These ANNs aim to replicate human cognitive processes by emulating the mechanisms observed in natural NNs. They serve the purpose of estimating or approximating functions that might rely on numerous inputs, many of which could be unfamiliar. ANNs are typically conceptualized as networks of interconnected neurons, facilitating the exchange of messages. Each connection possesses an associated weight, the value of which can be adjusted through learning from experience. This adaptive characteristic empowers NNs to accommodate diverse input types and facilitates their capacity to learn (Figure 6.1):
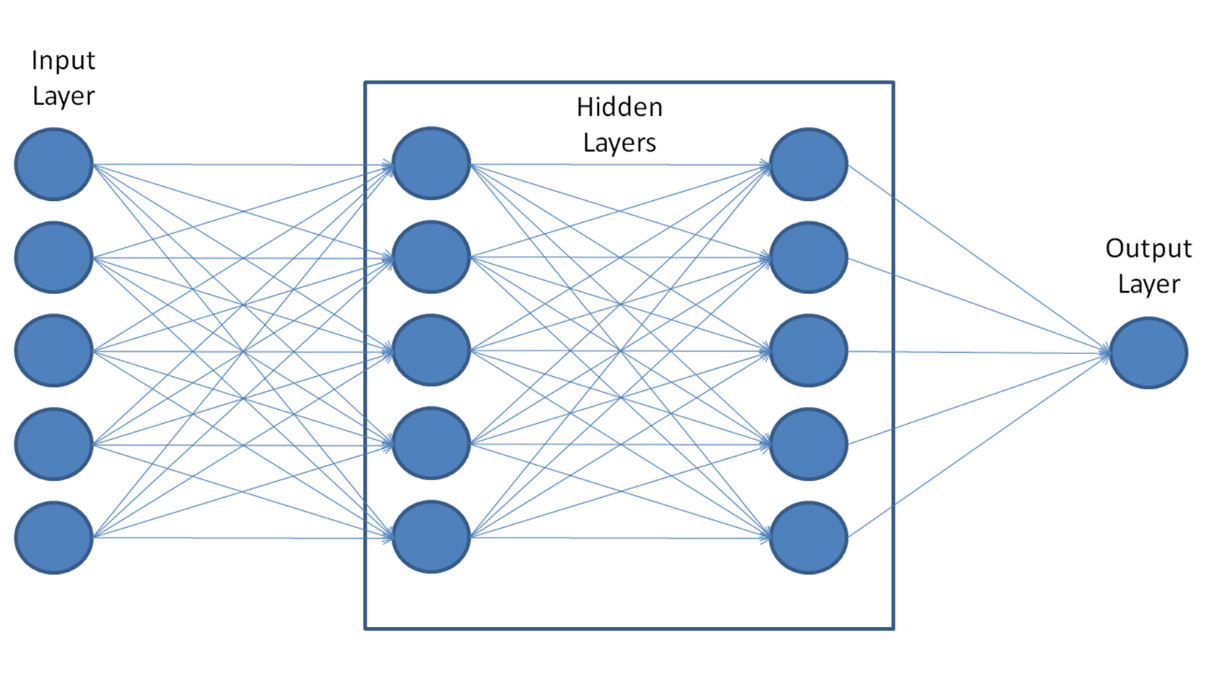
Figure 6.1 – ANN architecture with hidden layers
ANNs define a neuron as the central processing unit (CPU) that executes a mathematical operation to produce a single output from a set of input values. The neuron’...