In this chapter, we explored different forms of model-based architectures for one-shot learning. The most common thing we observed is the use of external memory, and how this can be helpful in learning representations at different stages of a neural network. NMT methods perform well on one-shot learning tasks, but they still have limited capacity because of hand-engineered memory-addressing functions, as they have to be differentiable. It might be interesting to explore more complex functions to address the memory. In meta networks, we saw how one new network was defined to enable fast learning of the original network, and how storing information about representations at the meta-learner level was useful to fine-tune parameters at the base level. Although model-based architectures are a good method of implementing one-shot learning, they come with the prerequisite of external...
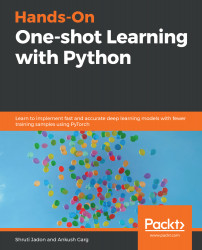
Hands-On One-shot Learning with Python
By :
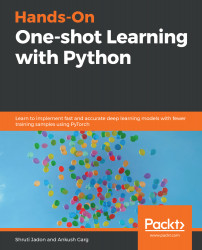
Hands-On One-shot Learning with Python
By:
Overview of this book
One-shot learning has been an active field of research for scientists trying to develop a cognitive machine that mimics human learning. With this book, you'll explore key approaches to one-shot learning, such as metrics-based, model-based, and optimization-based techniques, all with the help of practical examples.
Hands-On One-shot Learning with Python will guide you through the exploration and design of deep learning models that can obtain information about an object from one or just a few training samples. The book begins with an overview of deep learning and one-shot learning and then introduces you to the different methods you can use to achieve it, such as deep learning architectures and probabilistic models. Once you've got to grips with the core principles, you'll explore real-world examples and implementations of one-shot learning using PyTorch 1.x on datasets such as Omniglot and MiniImageNet. Finally, you'll explore generative modeling-based methods and discover the key considerations for building systems that exhibit human-level intelligence.
By the end of this book, you'll be well-versed with the different one- and few-shot learning methods and be able to use them to build your own deep learning models.
Table of Contents (11 chapters)
Preface
Section 1: One-shot Learning Introduction
Introduction to One-shot Learning
Section 2: Deep Learning Architectures
Metrics-Based Methods
Model-Based Methods
Optimization-Based Methods
Section 3: Other Methods and Conclusion
Generative Modeling-Based Methods
Conclusions and Other Approaches
Other Books You May Enjoy
Customer Reviews