Since we've had relatively good success using LBP in regards to texture recognition, let's try another example to understand the limitations of LBP. For this example, seven face colors from light to dark (test) will be matched with 10 foundation colors (train), which are images that are 50 x 50 in size.
Similar to the texture recognition examples, Steps 1 through 5 from the Generating an LBP pattern section will be applied and then each face color image LBP histogram will be compared with all of the foundation color image LBP histograms to find the best match. Although different histogram comparison methods have been used, for this analysis, the Chi-Square test will be used as the principal method for determining the match. The final summary output can be seen in the following image:
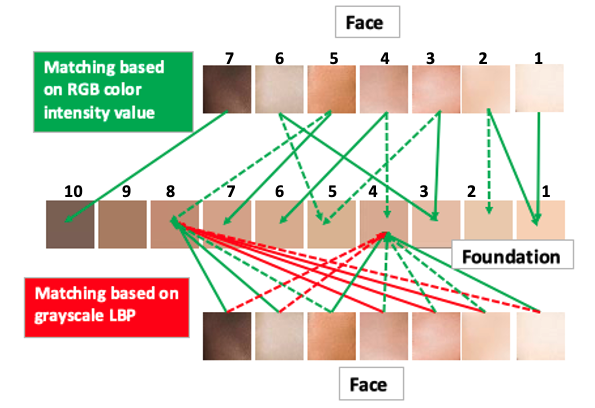
As we can...