Chapter 2
Introduction to Scikit-Learn and Model Evaluation
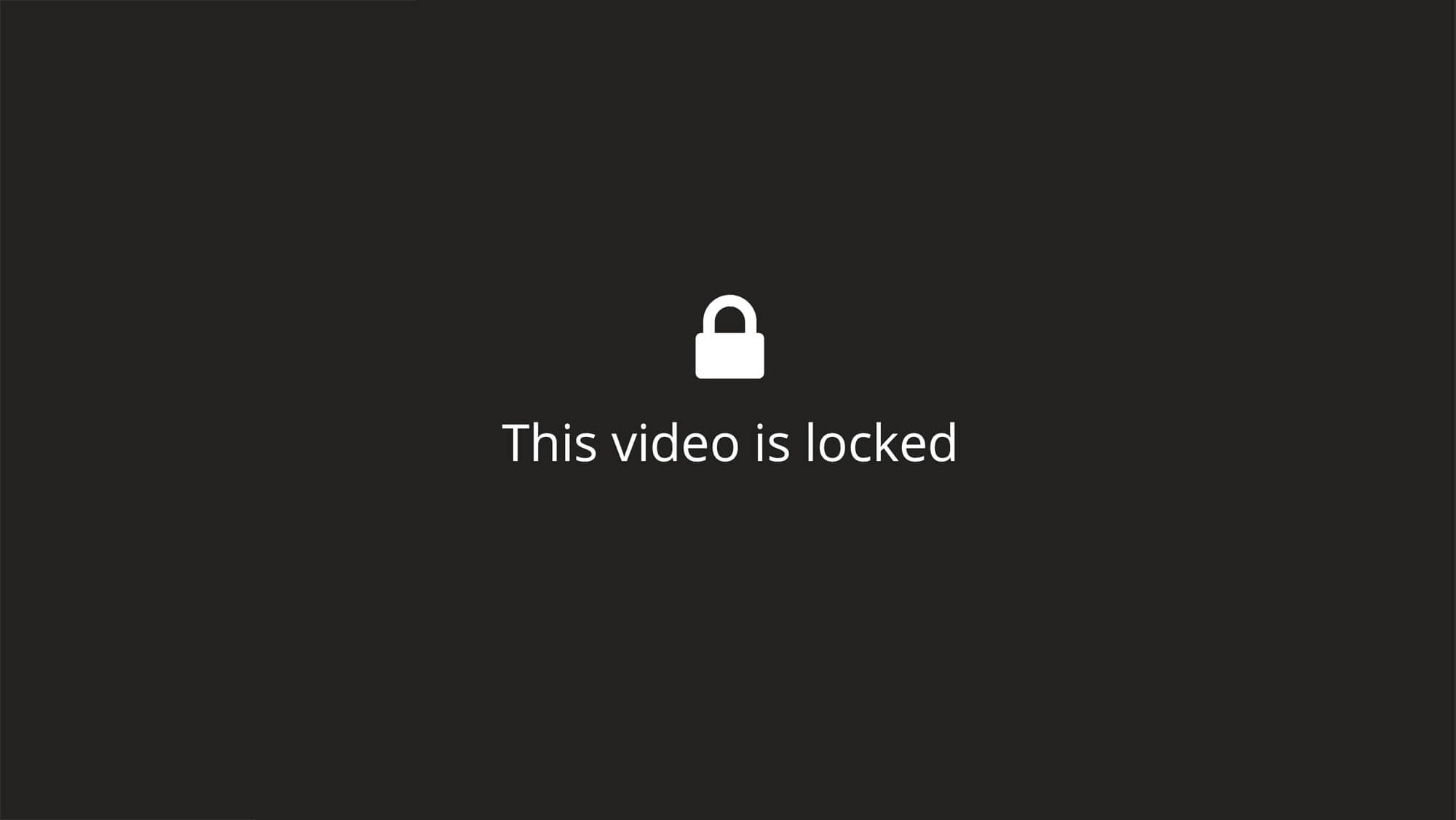
Section 5
True Positive Rate, False Positive Rate, and Confusion Matrix
In binary classification, there are just two labels to consider: positive and negative. As a more descriptive way to look at model performance than the accuracy of prediction across all samples, we can also look at the accuracy of only those samples that have a positive label. The proportion of these that we successfully predict as positive, is called the true positive rate (TPR). If we say that P is the number of samples in the positive class in the testing data, and TP is the number of true positives, defined as the number of positive samples that were predicted to be positive by the model, then the TPR is as follows: Here are the topics that we will cover now: