Chapter 6
Imputation of Missing Data, Financial Analysis, and Delivery to Client
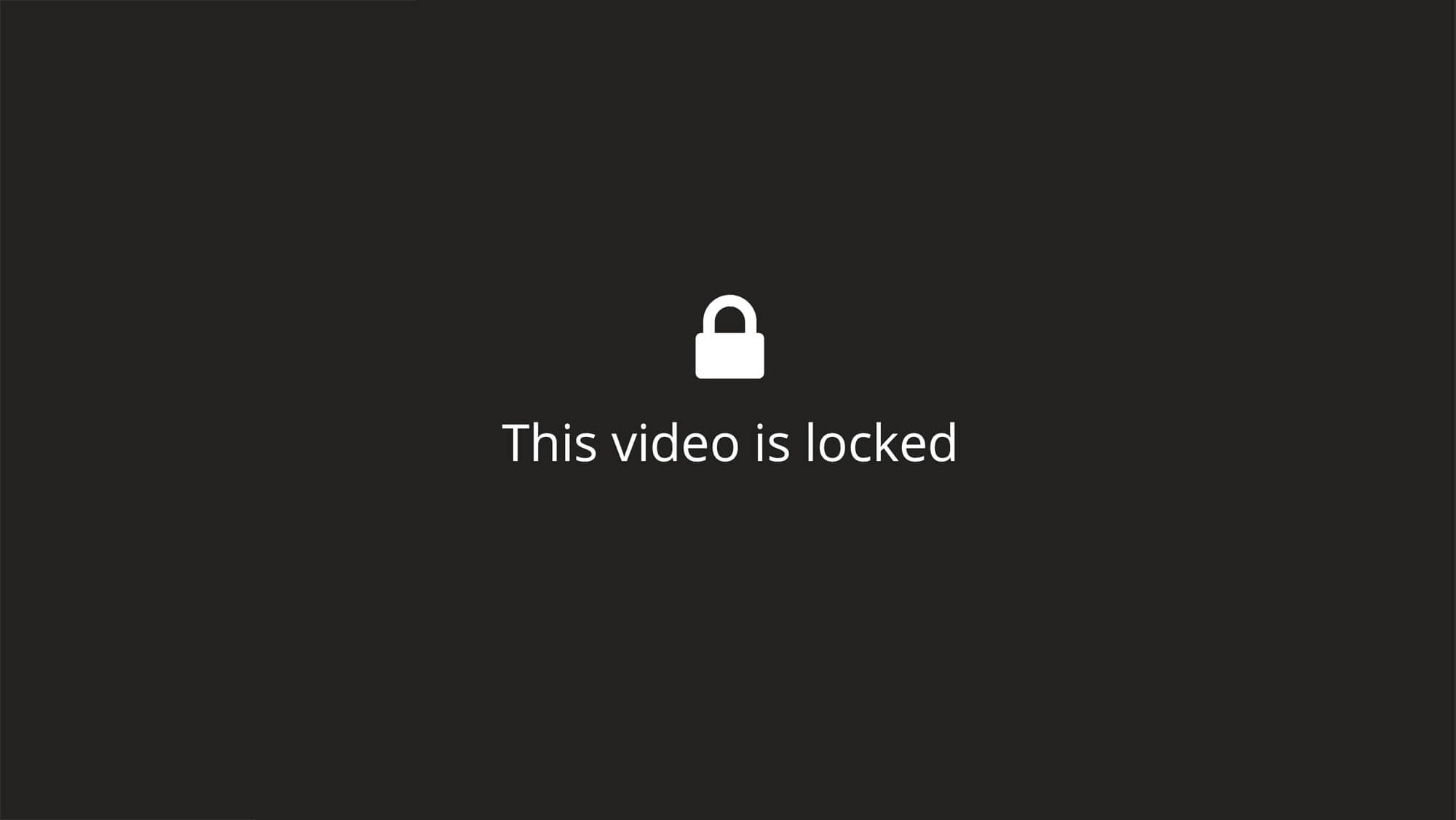
Section 8
Financial Analysis
The model performance metrics we have calculated so far were based on abstract measures that could be applied to analyze any classification model: how accurate a model is or how skillful a model is at identifying true positives relative to false positives (ROC AUC), or the correctness of positive predictions (precision). These metrics are important for understanding the basic workings of a model and are widely used within the machine learning community, so it's important to thoroughly understand them. However, for the application of a model to business processes, clients will not always be able to use such model performance metrics to establish an understanding of exactly how they will use a model to guide business decisions, or how much value a model can be expected to create. To go the extra mile and make the connection of the mathematical world of predicted probabilities and thresholds, to the business world of costs and benefits, a financial analysis of some kind is usually required. Here are the topics that we will cover now: