An interesting feature of a DQN is the utilization of a second network during the training procedure, which is referred to as the target network. This second network is used for generating the target-Q values that are used to compute the loss function during training. Why not use just use one network for both estimations, that is, for choosing the action a to take, as well as updating the Q-network? The issue is that, at every step of training, the Q-network's values change, and if we use a constantly changing set of values to update our network, then the estimations can easily become unstable – the network can fall into feedback loops between the target and estimated Q-values. In order to mitigate this instability, the target network's weights are fixed – that is, slowly updated to the primary Q-network's values. This...
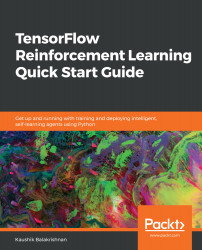
TensorFlow Reinforcement Learning Quick Start Guide
By :
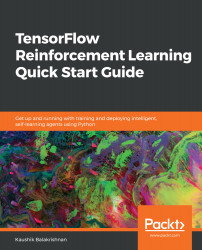
TensorFlow Reinforcement Learning Quick Start Guide
By:
Overview of this book
Advances in reinforcement learning algorithms have made it possible to use them for optimal control in several different industrial applications. With this book, you will apply Reinforcement Learning to a range of problems, from computer games to autonomous driving.
The book starts by introducing you to essential Reinforcement Learning concepts such as agents, environments, rewards, and advantage functions. You will also master the distinctions between on-policy and off-policy algorithms, as well as model-free and model-based algorithms. You will also learn about several Reinforcement Learning algorithms, such as SARSA, Deep Q-Networks (DQN), Deep Deterministic Policy Gradients (DDPG), Asynchronous Advantage Actor-Critic (A3C), Trust Region Policy Optimization (TRPO), and Proximal Policy Optimization (PPO). The book will also show you how to code these algorithms in TensorFlow and Python and apply them to solve computer games from OpenAI Gym. Finally, you will also learn how to train a car to drive autonomously in the Torcs racing car simulator.
By the end of the book, you will be able to design, build, train, and evaluate feed-forward neural networks and convolutional neural networks. You will also have mastered coding state-of-the-art algorithms and also training agents for various control problems.
Table of Contents (11 chapters)
Preface
Up and Running with Reinforcement Learning
Temporal Difference, SARSA, and Q-Learning
Deep Q-Network
Double DQN, Dueling Architectures, and Rainbow
Deep Deterministic Policy Gradient
Asynchronous Methods - A3C and A2C
Trust Region Policy Optimization and Proximal Policy Optimization
Deep RL Applied to Autonomous Driving
Other Books You May Enjoy
Customer Reviews