ML pipelines were developed to address the fact that machine learning is not just a bunch of algorithms, such as classification and regression, but a pipeline of actions performed over a Dataset. Let's take a quick look at the tasks involved in a typical machine learning process. The following figure shows the top-level activities:
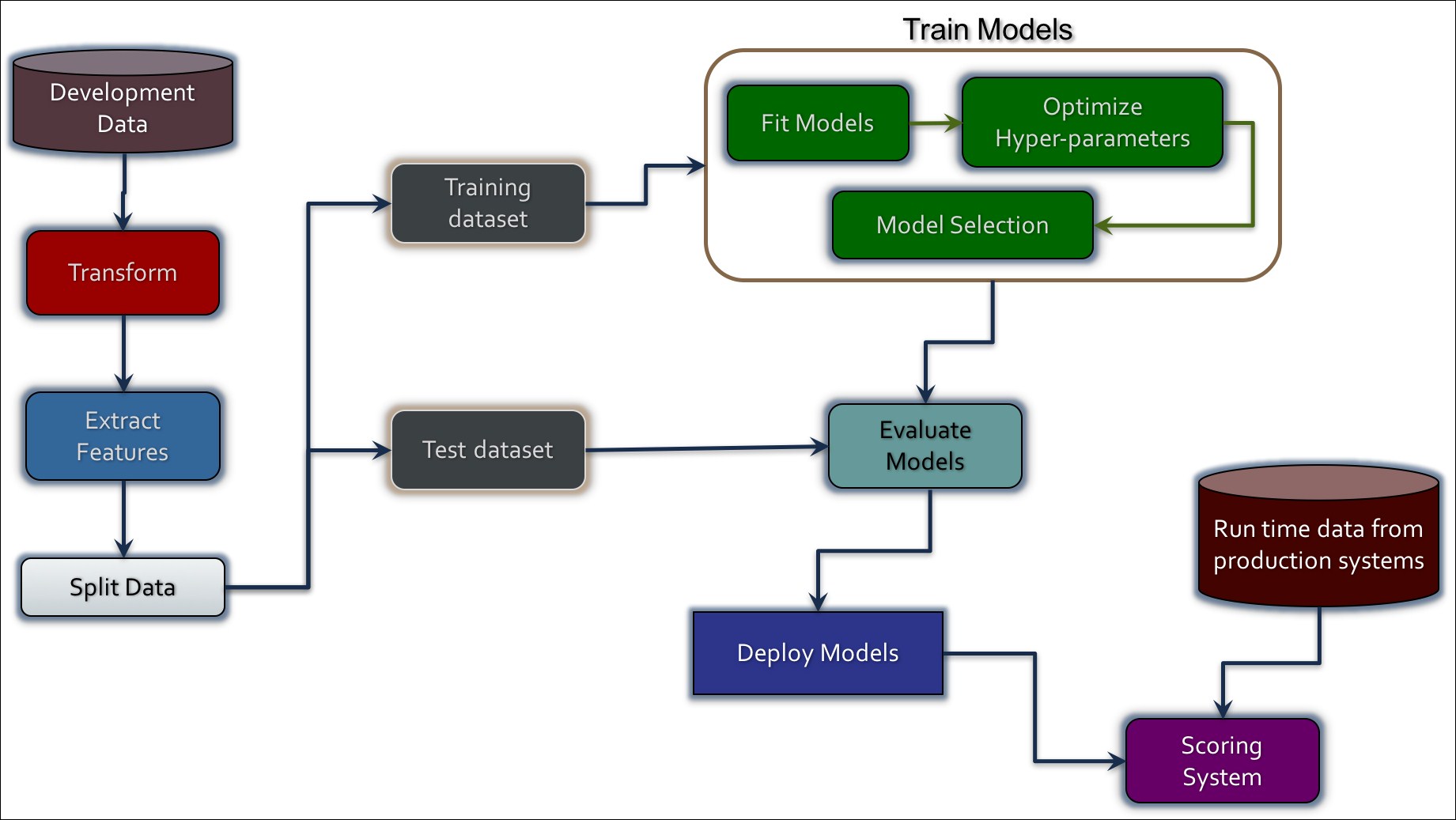
The first step is to get some data for the data science work. If you are using internal data, the data should be made anonymous and all PII information purged.
Once we have the data, we'll transform it: for example, we can convert a comma-separated CSV format into a DataFrame consisting of strings and numbers.
Then we extract the features that can be used to train our machine learning models. The feature extraction can be as simple as separating lines into words or normalizing words, such as deleting special characters and converting words to lowercase. This might also involve turning columns into categories, for example, Yes/No to 1/0 or Survived...