The biggest idea of all of the big ideas about TensorFlow is that the numeric computations are expressed as a computation graph, as shown in the following figure. So, the backbone of any TensorFlow program is going to be a computational graph, where the following is true:
- Graph nodes are operations which have any number of inputs and outputs
- Graph edges between our nodes are going to be tensors that flow between these operations, and the best way of thinking about what tensors are in practice is as n-dimensional arrays
The advantage of using such flow graphs as the backbone of your deep learning framework is that it allows you to build complex models in terms of small and simple operations. Also, this is going to make the gradient calculations extremely simple when we address that in a later section:
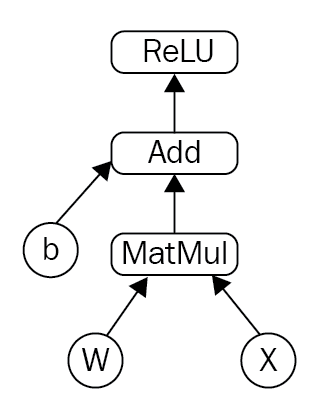
Another way of thinking about a TensorFlow graph is...