The visualization of the time series data has conveyed a similar story in all the examples, from Figure 1 to Figure 7. The trend and seasonality of the observations imply that the future values of the time series are dependent on the current values, and thus we can't assume that the observations are independent of each other. But what does this mean? To reiterate the point, consider the simpler uspop
(US population) dataset, the third-right panel display of Figure 7. Here, we don't have a seasonal influence. Now, consider the census year 1900. The population at the next census is certainly not less than in the year 1890, and it is not well beyond the same number of the same year. A similar narrative holds for most time series; for example, if we are recording the maximum temperature of the day. Here, if today's maximum temperature is 42°C, the next day's maximum temperature is highly influenced by this number and it is almost completely ruled out that the next day...
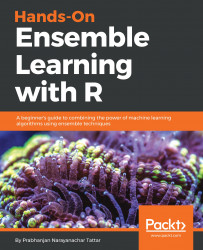
Hands-On Ensemble Learning with R
By :
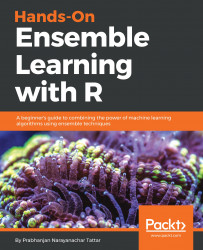
Hands-On Ensemble Learning with R
By:
Overview of this book
Ensemble techniques are used for combining two or more similar or dissimilar machine learning algorithms to create a stronger model. Such a model delivers superior prediction power and can give your datasets a boost in accuracy.
Hands-On Ensemble Learning with R begins with the important statistical resampling methods. You will then walk through the central trilogy of ensemble techniques – bagging, random forest, and boosting – then you'll learn how they can be used to provide greater accuracy on large datasets using popular R packages. You will learn how to combine model predictions using different machine learning algorithms to build ensemble models. In addition to this, you will explore how to improve the performance of your ensemble models.
By the end of this book, you will have learned how machine learning algorithms can be combined to reduce common problems and build simple efficient ensemble models with the help of real-world examples.
Table of Contents (17 chapters)
Hands-On Ensemble Learning with R
Contributors
Preface
Introduction to Ensemble Techniques
Bootstrapping
Bagging
Random Forests
The Bare Bones Boosting Algorithms
Boosting Refinements
The General Ensemble Technique
Ensemble Diagnostics
Ensembling Regression Models
Ensembling Survival Models
Ensembling Time Series Models
What's Next?
Bibliography
Index
Customer Reviews