The tree-based ensembles in the previous chapters, Bagging and Random Forests, cover an important extension of the decision trees. However, while bagging provides greater stability by averaging multiple decision trees, the bias persists. This limitation motivated Breiman to sample the covariates at each split point to generate an ensemble of "independent" trees and lay the foundation for random forests. The trees in the random forests can be developed in parallel, as is the case with bagging. The idea of averaging over multiple trees is to ensure the balance between the bias and variance trade-off. Boosting is the third most important extension of the decision trees, and probably the most effective one. It is again based on ensembling homogeneous base learners (in this case, trees), as are the bagging and random forests. The design of the boosting algorithm is completely different though. It is a sequential ensemble method in that the residual/misclassified...
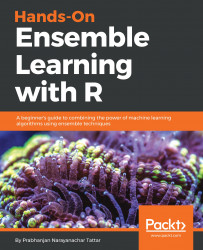
Hands-On Ensemble Learning with R
By :
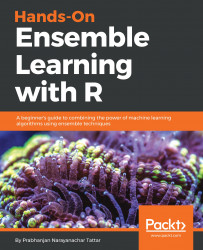
Hands-On Ensemble Learning with R
By:
Overview of this book
Ensemble techniques are used for combining two or more similar or dissimilar machine learning algorithms to create a stronger model. Such a model delivers superior prediction power and can give your datasets a boost in accuracy.
Hands-On Ensemble Learning with R begins with the important statistical resampling methods. You will then walk through the central trilogy of ensemble techniques – bagging, random forest, and boosting – then you'll learn how they can be used to provide greater accuracy on large datasets using popular R packages. You will learn how to combine model predictions using different machine learning algorithms to build ensemble models. In addition to this, you will explore how to improve the performance of your ensemble models.
By the end of this book, you will have learned how machine learning algorithms can be combined to reduce common problems and build simple efficient ensemble models with the help of real-world examples.
Table of Contents (17 chapters)
Hands-On Ensemble Learning with R
Contributors
Preface
Introduction to Ensemble Techniques
Bootstrapping
Bagging
Random Forests
The Bare Bones Boosting Algorithms
Boosting Refinements
The General Ensemble Technique
Ensemble Diagnostics
Ensembling Regression Models
Ensembling Survival Models
Ensembling Time Series Models
What's Next?
Bibliography
Index
Customer Reviews